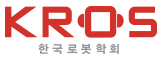
행동 인식을 위한 시공간 앙상블 기법
© Korea Robotics Society. All rights reserved.
Abstract
As deep learning technology has been developed and applied to various fields, it is gradually changing from an existing single image based application to a video based application having a time base in order to recognize human behavior. However, unlike 2D CNN in a single image, 3D CNN in a video has a very high amount of computation and parameter increase due to the addition of a time axis, so improving accuracy in action recognition technology is more difficult than in a single image. To solve this problem, we investigate and analyze various techniques to improve performance in 3D CNN-based image recognition without additional training time and parameter increase. We propose a time base ensemble using the time axis that exists only in the videos and an ensemble in the input frame. We have achieved an accuracy improvement of up to 7.1% compared to the existing performance with a combination of techniques. It also revealed the trade-off relationship between computational and accuracy.
Keywords:
Action Recognition, 3D CNN, Deep Learning1. 서 론
현대의 Deep Convolutional Neural Networks (CNNs)는 다양한 컴퓨터 비전 분야에서 놀라운 발전을 이루어왔다. 예를 들어 ImageNet Large Scale Visual Recognition Challenge (ILSVRC)[1]와 같은 이미지 분류 대회에서는 ResNet[2]의 등장으로 사람의 이미지 분류 정확도를 뛰어 넘었다.
그밖에도 CNNs는 super resolution[3], object detection[4], semantic segmentation[5] 등 다양한 딥러닝 분야에서 좋은 결과를 보여주고 있다. 이러한 딥러닝의 발전은 독립된 하나의 프레임에서 주로 이루어져 왔다. 하지만 여러 장의 이미지가 합쳐진 동영상에서의 CNNs도 현대의 딥러닝 기반의 어플리케이션에서 필수적인 기술이다.
여러 장의 이미지가 합쳐진 동영상은 2D CNNs에서 시간축의 차원이 증가하여 3D CNNs를 구성한다. 따라서 축이 하나 증가하였기 때문에 연산량과 매개변수들의 개수가 매우 높아, 충분한 메모리가 보장된 일부 고성능의 GPU에서만 연구가 가능하다. 또한 ImageNet과 같은 대규모의 정제된 데이터셋이 없었기 때문에 행동 인식에 대한 연구를 진행하기 어려웠지만 최근 DeepMind에서 공개한 Kinetics[6] 데이터셋의 공개로 활발하게 진행되고 있다.
Kinetics 데이터셋이 공개된 현재에도 해결해야 할 문제점은 남아있다. 행동 인식의 네트워크는 매개변수가 매우 높아 과적합 문제가 빈번히 발생한다. 이러한 과적합 문제를 해결하기 위하여 연구자들은 Kinetics와 같은 대규모 데이터셋을 사용하여 과적합 문제를 해결하려고 한다. 그러나 해당 대규모 데이터셋에 대하여 I3D (Inflated 3D ConvNet)[6] 네트워크 구조를 처음부터 학습 할 경우에는 Nvidia사의 V100 GPU 16장이 탑재된 머신을 사용하였을 때, 대략 2주 정도의 긴 시간이 걸린다. 따라서 Kinetics 데이터셋을 처음부터 학습시킬 환경을 구성하지 못하는 경우에는 사전에 공개된 Kinetics 학습 모델에 의존적이 된다. 또한 네트워크의 구조를 변경하면 Kinetics 사전 학습 모델을 사용할 수 없다. 따라서 우리는 이 문제들을 해결하기 위해 네트워크 구조의 변경 없이 3D CNN 기반의 행동인식 모델에서 성능을 개선하는 방법들을 조사하고 비교한다.
첫 번째로 우리는 행동인식 모델에서 테스트 시 입력 프레임을 조절하는 방법을 제안한다. 기존의 3D CNN 비디오 모델은 mini-batch 크기를 구성하는 B × T × H × W (mini batch size × number of frames × height × width) 수치들이 고정된 상태로 학습이 진행되고 테스트 시에도 마찬가지로 학습 때 사용했던 고정 된 mini batch 크기가 사용된다.
하지만 3D CNN은 학습하는 동영상들의 길이(1~10초)가 모두 달라도 고정된 값 T를 사용한다. 따라서 고정된 T 때문에 3D CNN으로 학습되는 동영상들은 T의 길이가 모든 동영상의 모든 부분을 포함할 정도로 충분할 수도, 반대로 불충분할 수도 있다. 예를 들어 [Fig. 1]과 같이 25 FPS로 비디오에서 프레임을 추출했으면, 위의 동영상과 같이 3.5초면 84개의 프레임이 추출되고, 아래와 같이 10초짜리 동영상의 경우에는 224개의 프레임이 추출된다. 하지만 3D CNN에서는 고정된 T의 길이를 사용하기 때문에, T의 길이를 128로 고정하였다면 위의 동영상은 충분한 T의 길이를 가지고 있는 것이고 아래의 동영상은 충분한 T의 길이를 가지지 못하여 일부의 부분만 학습에 사용되는 것이다. 따라서 이러한 환경에서 학습된 3D CNN 모델은 동영상의 길이에 강인하게 학습된다.
![[Fig. 1] [Fig. 1]](/xml/26775/JKROS_2020_v15n4_385_f001.jpg)
A typical video model has a fixed mini-batch size even if the lengths of the images are all different, as shown in the figure. For this reason, the mini-batch size extracted as shown on the top of the picture may include the entire image, or may not include the entire images as shown below. Therefore, the video recognition model trained in a fixed mini-batch size regardless of the overall size of the image is strongly trained in changing the frame
이러한 단점을 극복하기 위하여, 고정된 mini-batch 크기에서 학습된 모델을 평가할 때 서로 다른 프레임의 개수로 평가하는 방법을 제안한다. 예를 들어 학습할 때에는 mini-batch 크기가 16 × 64 × 112 × 112로 고정되었다면 테스트할 때에는 16 × 128 × 112 × 112, 16 × 250 × 112 × 112 등 다양한 T의 길이로 평가하여 입력 동영상의 프레임 개수 차이에 대응한다.
두 번째로 우리는 입력에서의 앙상블 기법을 제안한다. 보통 앙상블 기법은 하나의 동일한 네트워크 구조에서 서로 각기 다른 네트워크 초기화 상태, 즉 매개변수의 처음 시작 지점이 각기 다른 상태의 네트워크들을 사용한다. 이렇게 추출된 여러 개의 네트워크들에 동일한 입력을 넣고 각기 다른 매개변수를 가지고 있는 같은 네트워크에서 출력된 다양한 출력을 앙상블하고 최종 출력으로 사용한다. 하지만 앞에서 언급한 것과 같이 3D CNN 기반의 행동 인식 모델은 학습 시간과 컴퓨터 자원을 많이 소모하므로 기존 방식의 앙상블 방법은 모델을 여러 개 보유하고 있어야하기 때문에 매우 비효율적이다. 따라서 우리는 하나의 동일한 모델에서 하나의 동영상에 대해 다양한 기하학적 데이터 변형을 적용하여 생성된 변화된 여러 가지 동영상을 입력으로 주고 출력된 다양한 결과를 앙상블한다. 이 방법은 추가적인 네트워크의 사용 없이 앙상블 기법을 적용하는 방법으로 다양한 네트워크를 학습을 시킬 필요가 없다. 본 논문에서는 제안한 방식에 대한 효과적인 검증을 위하여 행동인식 연구 분야에 대표적으로 사용되는 HMDB-51[7]와 3D-ResNeXt101[8]을 활용하여 성능을 입증한다.
2. 관련 연구
2.1 2D CNN기반의 행동인식 모델
초기의 행동 인식 연구는 2D CNN 네트워크를 활용하여 행동인식의 비디오를 입력으로 활용하여 진행되었다. 이에 대한 방법은 각 프레임에서 독립적으로 특징을 추출하고 모든 특징을 융합하여 예측하는 것이다[9]. 하지만 이러한 2D CNN을 기반으로 한 구현은 간편하지만 시간적인 순서를 완전히 무시하는 문제가 있었다. (예 : 사람이 문을 열고 나가는 것과 문을 닫고 들어오는 것을 구별할 수 없음.) 시간적 순서를 무시하는 현상을 극복하기 위하여 이후에 특징을 뽑고 시간 순서와 장거리 종속성을 캡처 할 수 있는 LSTM과 같은 RNN을 모델에 추가하였다[10,11]. 초기모델에 비해 성능은 향상이 되었지만, 이러한 방식들 또한 여전히 시간적 순서를 잘 포착하지 못한다는 문제를 가지고 있었다. 이러한 이유로 높은 정확도를 달성한 최신 방법들의 모델들은 더 이상 2D CNN과 LSTM의 조합으로 행동인식 모델을 구성하지 않는다. 따라서 우리의 연구도 2D CNN의 구조를 가진 네트워크는 제외하고 실험하였다.
2.2 3D CNN 기반의 행동인식 모델
3D CNN은 비디오 네트워크 모델링에 대하여 가장 직관적인 방법 중 하나로, 기존 2D CNN와 비슷하지만 시간축이 추가된 시공간 필터를 사용한다.
이러한 방법들은 이전에 여러 차례 연구 되었다[12-15]. 3D CNN 모델의 한 가지 문제는 추가 커널 차원으로 인해 2D CNN보다 많은 매개변수가 존재하므로 과적합이 발생하기 쉽고, 학습하기가 더 어렵다. 또한 시간축이 추가되었기 때문에 ImageNet 사전 학습 모델의 이점을 사용할 수 없다. 따라서 초기의 3D CNN의 구조는 상단에 8개의 convolution layer, 5개의 pooling Layer 및 2개의 fully connected layer 구성으로, 과적합을 방지하기 위해 비교적 연산량이 적은 네트워크 구조에 기반을 두고 연구가 진행이 되었다.
3D CNN는 발전 가능성을 보여 주었지만 당시의 optical flow를 사용한 네트워크 대비 성능이 유망하지 않기 때문에 더 큰 데이터에서의 학습이 된 사전 학습 모델이 필요했다. 이후 대규모 데이터셋인 Kinetics 데이터셋이 공개되고 사전 학습 모델을 활용하여 네트워크를 더욱 깊게 설계할 수 있었다.
이후에 나온 3D CNN의 대표인 모델의 I3D는 ImageNet으로 사전 학습된 커널을 시간 축으로 확장하여 높은 정확도를 달성하였다. 그 이외에도 2D CNN에서 잘된다고 알려진 구조들이 3D CNN에서도 잘된다고 실험적으로 보인 연구들이 나오고 3D CNN은 행동인식에서 주류가 되었다. 따라서 우리는 3D CNN에서만 작동하는 방법을 제안하고 실험하였다.
3. 연구 방법
이 부분에서는 우리가 제안한 기존 앙상블 기법에 대한 효과를 낼 수 있는 입력 동영상에 대한 공간적 앙상블 기법과 시간적 앙상블 기법을 제안하고 검증한다.
3.1 입력 동영상들의 공간적 앙상블
일반적으로 CNN모델에서 사용하는 앙상블 기법들은 같은 모델, 데이터셋에서 동일한 학습 기법으로 학습시켜 여러 개의 모델을 만들고 모든 출력 결과에 대한 평균을 구하거나, 투표하는 방식으로 성능을 향상하는 기법이다. 이 기법은 2D CNN에서 성능 향상을 위해 많이 사용된다. 하지만 [Fig. 2]의 그림과 같이 3D CNN에서 성능 향상을 위해 동일한 방법을 쓸 경우 성능 향상 대비 파라메터, 연산량이 매우 크게 증가하여 자주 사용되지 않는다. 그렇기 때문에 3D CNN에서 파라메터와, 연산량에 제한을 받지 않는 앙상블 기법은 행동인식 분야에서 필요한 기술이다.
우리는 이러한 문제점을 해결하기 위하여 입력 동영상들의 공간적 앙상블을 제안한다. [Fig. 3]의 그림과 같이 우리가 제안하는 입력 동영상 앙상블의 방법은 한 번의 학습만으로 만들어진 하나의 모델에서 같은 동영상이지만 기하학적으로 변형을 준 이미지가 입력으로 들어가게 되며 여러 개의 출력을 만들어 준다. 기하학적 변형을 준 입력에서 추출된 모든 결과를 평균 또는 투표를 사용하여 앙상블 하면 기존의 앙상블과 비슷한 효과를 기대할 수 있다.
3.2 입력 동영상들의 시간적 앙상블
단일 이미지 기반의 2D CNN모델과는 다르게 동영상에서의 3D CNN은 시간 축이 존재한다. 따라서 우리는 비디오에서 가지고 있는 시간적 특징을 활용하여 테스트 시에 적용할 수 있는 시간적 앙상블을 제안한다.
우리가 제안한 시간적 앙상블은 일반적인 3D CNN과 같이 고정적인 mini-batch 크기로 학습을 진행한다. 하지만 테스트 시에는 고정적인 mini-batch 크기가 아닌, 프레임의 개수를 조절하여 T를 동적으로 변경한다. 예를 들어 학습 시에 mini-batch shape가 1 × 64 × 112 × 112의 고정적 크기를 가지고, 테스트 시에는 1 × T × 112 × 112 의 크기를 가지고 T 값을 다양하게 조절한 뒤, 동일 비디오의 다양한 결과 값을 만들어 결합하여 사용한다.
시간적 앙상블은 하나의 모델에서 다양한 mini-batch 크기가 입력된 후 추출되는 모든 특징은 같은 크기로 만들어 주어야 한다. 이러한 이유로 인해 기존 네트워크에서 간단한 수정이 있으면 된다. [Fig. 4]의 그림과 같이 기존의 네트워크에 adaptive average pooling layer를 추가하면 feature extractor를 통과하여 나온 모든 특징들은 mini-batch 크기와는 관계없이 늘 고정적인 모양이 나온다.
4. 실 험
동작 인식 벤치마크 데이터셋 중 하나인 HMDB-51의 Split.1에서 우리가 제안한 앙상블 기법들을 평가했다. HMDB-51은 51개의 동작 범주로 구성되며 포함된 총 클립의 수는 약 7,000개다. 우리는 평가를 위해 3D-ResNeXt-101를 사용했으며, RGB 이미지뿐만 아니라 TV-L1[16] 알고리즘으로 추출 된 optical flow를 평가에 사용하였다. 우리의 모든 실험 환경은 MARS[17]의 실험 환경과 동일하게 설정을 하였다.
우리는 첫 번째로 테스트 시 프레임의 개수와 성능사이의 trade-off 관계를 분석하기 위하여 Kinetics-400에서 학습된 사전학습 모델을 사용하여 HMDB-51에서 미세 조정하였으며, 우리가 학습시킨 3D-ResNeXt-101의 mini-batch 크기는 16 × 64 × 112 × 112로 고정된다.
[Table 1]은 위와 같은 조건으로 학습된 사전 학습 모델을 사용하여 테스트한 결과이다. 테스트 프레임의 값은 32, 64, 128, 256을 사용한다. [Table 1]에 보이는 결과와 같이 고정된 mini-batch 크기로 학습된 사전 학습 모델 이지만 mini-batch 크기를 변경하더라도 성능에 미치는 영향이 크지 않음을 실험적으로 증명했다. 또한 RGB 동영상에서 테스트 프레임 개수가 많을수록 성능이 향상됨을 보였다. 하지만 프레임 개수가 늘어날수록 메모리와 연산량이 증가하였다. 어플리케이션에 이용하기 위해서는 해당 어플리케이션에서 요구하는 실시간성과 성능사이의 trade-off 관계를 확인하고 프레임의 개수를 조절하면 된다.
![[Table 1]](../img/npr_tablethum.jpg)
3D ResNeXt 101 performance in HMDB51 action recognition benchmark. 3 channels of RGB modality are input. modalities stacked 32,64,128,250 consecutive frames, and the spatial size of the image is (112,112). All performance is the result of fine-tuning the pre-trained weight of Kinetics400
[Table 2]의 결과는 TV-L1알고리즘으로 추출된 optical flow가 입력될 때 입력 프레임의 개수와 성능사이에 관계가 없음을 보인다. 이를 통해서 테스트에서 optical flow modality를 사용하면 최소한의 프레임을 입력으로 사용할 수 있었으며, 연산량과 메모리에서 효율적이라는 것을 실험적으로 밝혔다.
![[Table 2]](../img/npr_tablethum.jpg)
3D ResNeXt 101 performance in HMDB51 action recognition benchmark. 2 channels of Flow modality are input. modalities stacked 32,64,128,250 consecutive frames, and the spatial size of the image is (112,112). All performance is the result of fine-tuning the pre-trained weight of Kinetics400
우리는 마지막으로 입력 동영상에서의 앙상블 성능의 확인을 위하여 첫 번째에서의 실험과 동일한 환경에서 실험 하였다. 제안한 방법에 대한 실험은 모든 기하학적 변형을 하나의 동영상의 모든 프레임에 대하여 동일하게 사용되었다. 예를 들어 입력으로 들어가는 첫 번째 프레임에서 좌우 반전이 사용되었으면, 마지막 프레임까지 모두 좌우 반전되어 입력으로 사용되었다.
[Table 3]의 결과는 수평 반전이 적용된 동영상의 앙상블은 성능이 상승하였지만 수직 반전이 적용된 동영상의 앙상블은 성능이 하락하였다. 수직 반전 동영상의 성능이 저하한 이유는 현실에서 존재하지 않는 데이터(사람의 얼굴이 땅을 향하고, 다리가 하늘을 향하는 현실에서 존재하기 힘든 영상. )가 생성되어 학습에도 사용하지 않으며, 테스트시 모델의 입력으로 들어가면 행동인식 네트워크가 잘못된 방향으로 결과를 도출하기 때문이다. 따라서 기하학적 데이터 증식 방법은 테스트 데이터셋에서 존재할 수 있는 수준의 변형만 수행도록 하였다. 따라서 우리의 실험에서는 수직 반전은 제외한다.
![[Table 3]](../img/npr_tablethum.jpg)
3D ResNeXt 101 performance in HMDB51 action recognition benchmark. 3 channels of RGB modality are input. All performance is the result of fine-tuning the pre-trained weight of Kinetics400. Horizontal is the image with horizontal flip applied to the input image. Lastly, Vertical is an image with vertical flip applied
[Table 4]의 결과는 전체 프레임에서 각 프레임을 개별적으로 다른 데이터증강 방법을 적용한 것과 전체 프레임에서 전체 프레임에 대하여 모두 동일한 데이터 증강을 적용한 것의 비교이다. [Table 4]의 표에서도 보이는 것과 같이, 전체 동영상에 걸쳐서 모두 동일한 데이터 증강을 적용하여야 성능이 오르는 것을 확인할 수 있었다. 이와 같은 실험결과를 바탕으로 우리가 제안한 방법에 대한 실험에서는 모든 프레임에서 동일한 데이터 증강을 적용하였다.
![[Table 4]](../img/npr_tablethum.jpg)
3D ResNeXt 101 performance in HMDB51 action recognition benchmark. 3 channels of RGB modality are input. All performance is the result of fine-tuning the pre-trained weight of Kinetics400. ‘Single’ is augmentation applied to the input image every single frame, and ‘All’ is the same augmentation applied to all frames. Also, all augmentation is horizontal flip
우리는 본격적으로 입력 동영상에서의 앙상블을 실험하기 위하여, [Table 2]의 실험에서 분석한 결과를 바탕으로 수평 반전과 영상 일부 자르기 등의 데이터 증강 방법들을 채택하였으며, [Table 4]의 결과를 바탕으로 데이터 증강은 동일한 동영상의 모든 프레임에 대해서 동일한 데이터 증강 방법을 적용하였다. [Fig. 5]의 그림은 우리의 실험에 사용된 데이터 증강의 예시이다. 수평 반전과 영상 일부 자르기는 서로 조합이 가능하기 때문에 우리가 실험한 것 이상의 조합의 수가 나오지만, 우리의 방법으로 인한 성능 향상을 확인하기 위해서는 6개의 모델의 앙상블도 충분하다고 판단하였다.
![[Fig. 5] [Fig. 5]](/xml/26775/JKROS_2020_v15n4_385_f005.jpg)
The above picture is an image of horizontal filp, left-top crop, right-top crop, left-down crop, and right-down crop of the original picture to ensemble the input. In addition, horizontal flip can be combined with each crop method, so a total of 6 combinations can be made
[Table 5]의 표는 우리의 입력 동영상에서의 앙상블에 대한 결과이다. 표에서 보이는 것과 같이 입력 동영상의 앙상블의 개수가 많아질수록 성능이 향상되는 것을 실험적으로 확인할 수 있었다. 입력 동영상에서의 앙상블을 통하여 매개변수의 증가 없이 성능은 향상되지만, 연산량의 증가로 인해서 실시간성은 떨어졌다. 정확도가 중요한 어플리케이션이라면 입력 동영상에서의 앙상블 모델의 개수를 늘려서 원하는 정확도까지 늘려서 사용하는 것이 좋다. 이와 반대로 실시간성이 중요한 어플리케이션이라면 입력 동영상에서의 앙상블을 사용하지 않는 것이 좋다는 것을 실험적으로 보였다.
![[Table 5]](../img/npr_tablethum.jpg)
3D-ResNeXt-101 performance in HMDB-51 action recognition benchmark. 3 channels of RGB modality are input. All performance is the result of fine-tuning the pre-trained weight of Kinetics400. In the surrender of “Combination of Ensembles,” ‘1’ represents the original image, and ‘2’ is the horizontal filp. 3 to 6 are left-top, right-top, left-down, and right-down crops sequentially
또한 우리는 시간축의 앙상블과 입력 동영상에서의 앙상블의 조합으로 같은 모델에서 성능을 최대 얼마나 상승시킬 수 있는지 확인하였다. 이를 실험적으로 증명하기 위하여 시간축의 앙상블 최고 성능 결과와 입력 동영상에서의 앙상블 최고 성능 결과를 동시에 사용하였다. [Table 6]은 그에 대한 실험 결과이다. [Table 6]에 보이는 것과 같이 우리의 방법을 사용하면, 학습 모델을 변경하지 않아도 최대 7.1%의 성능이 향상될 수 있음을 확인할 수 있었다.
![[Table 6]](../img/npr_tablethum.jpg)
3D-ResNeXt-101 performance in HMDB-51 action recognition benchmark. 3 channels of RGB modality are input. All performance is the result of fine-tuning the pre-trained weight of Kinetics400. Temporal is the time axis with the best performance of 250f, and spatial is the ensemble of six input images
최종적으로 우리가 제안한 앙상블 방법을 기존의 다중 모델 앙상블 방법과 비교해 보았다. [Table 7]에서 보여주는 것과 같이 우리가 제안하는 방법의 앙상블은 파라메터 증가 없이 기존 다중 모델 앙상블 모델 앙상블과 비슷한 성능을 보였다. 우리의 앙상블 방법의 연산속도는 기존의 앙상블과 같이 선형적으로 증가하지만, 파라메터의 증가가 없어 메모리가 부족한 GPU 환경에서 메모리의 제약 없이 높은 성능을 달성하는 방법이다.
5. 결 론
우리는 행동인식에 특화된 새로운 앙상블 방법을 제안하였다. 우리의 방법은 학습된 하나의 모델에서 입력 프레임 개수조절과 입력 동영상의 데이터 증강으로 추가적인 학습 없이 성능을 올리는 방법이다. 행동인식의 성능을 향상시켜야 하지만, 대용량 연산 자원의 필요로 인해 성능을 향상 시키지 못하는 산업에서 유용할게 사용 될 수 있을 것이다. 결과적으로, 우리가 제안한 방법을 사용하면 기존에 학습된 하나의 단일 모델에서 성능을 최대 7.1% 향상 시킬 수 있었다.
Acknowledgments
This research was supported by the National Research Foundation of Korea (NRF) funded by the Ministry of Science and ICT (NRF-2018R1C1B5086502, NRF-2020R1F1A1076557)
References
-
O. Russakovsky, J. Deng, H. Su, J. Krause, S. Satheesh, S. Ma, Z. Huang, A. Karpathy, A. Khosla, M. Bernstein, A. C. Berg, and Li Fei-Fei, “Imagenet large scale visualrecognition challenge,” International Journal of Computer Vision, vol. 115, pp. 211-252, 2015.
[https://doi.org/10.1007/s11263-015-0816-y]
-
K. He, X. Zhang, S. Ren, and J. Sun, “Deep residual learning for imagerecognition,” 2016 IEEE Conference on Computer Vision and Pattern Recognition (CVPR), Las Vegas, NV, USA, pp. 770-778, 2016.
[https://doi.org/10.1109/CVPR.2016.90]
-
C. Dong, C. C. Loy, K. He, and X. Tang, “Image super-resolution using deepconvolutional networks,” IEEE Transactions on Pattern Analysis and Machine Intelligence, vol. 38, no. 2, pp. 295-307, Feb., 2015.
[https://doi.org/10.1109/TPAMI.2015.2439281]
-
S. Ren, K. He, R. Girshick, and J. Sun, “Faster r-cnn: Towards real-time object detection with region proposal networks,” IEEE Transactions on Pattern Analysis and Machine Intelligence, vol. 39, no. 6, pp. 1137-1149, Jun., 2017.
[https://doi.org/10.1109/TPAMI.2016.2577031]
-
L.-C. Chen, Y. Zhu, G. Papandreou, F. Schroff, and H. Adam, “Encoder-decoder with atrous separableconvolution for semantic image segmentation,” European Conference on Computer Vision, pp. 833-851, 2018.
[https://doi.org/10.1007/978-3-030-01234-2_49]
-
J. Carreira and A. Zisserman, “Quo vadis, action recognition?,” 2017 IEEE Conference on Computer Vision and Pattern Recognition (CVPR), Honolulu, HI, USA, 2017.
[https://doi.org/10.1109/CVPR.2017.502]
-
H. Kuehne, H. Jhuang, E. Garrote, T. Poggio, and T. Serre, “HMDB: a large video database forhuman motion recognition,” 2011 International Conference on Computer Vision, Barcelona, Spain, 2011.
[https://doi.org/10.1109/ICCV.2011.6126543]
-
K. Hara, H. Kataoka, and Y. Satoh, “Can spatiotemporal 3d cnns retrace the history of 2d cnns and imagenet?,” 2018 IEEE/CVF Conference on Computer Vision and Pattern Recognition, 2018.
[https://doi.org/10.1109/CVPR.2018.00685]
-
A. Karpathy, G. Toderici, S. Shetty, T. Leung, R. Sukthankar, and L. Fei-Fei, “Large-scale video classification with convolutional-neural networks,” 2014 IEEE Conference on Computer Vision and Pattern Recognition, 2014.
[https://doi.org/10.1109/CVPR.2014.223]
-
J. Yue-Hei Ng, M. Hausknecht, S. Vijayanarasimhan, O.Vinyals, R. Monga, and G. Toderici, “Beyond short snippets: Deep networks for video classification,” 2015 IEEE Conference on Computer Vision and Pattern Recognition (CVPR), Boston, MA, USA, 2015.
[https://doi.org/10.1109/CVPR.2015.7299101]
-
J. Donahue, L. A. Hendricks, S. Guadarrama, M. Rohrbach, S. Venugopalan, T. Darrell, and K. Saenko, “Long-term recurrentconvolutional networks for visual recognition and description,” 2015 IEEE Conference on Computer Vision and Pattern Recognition (CVPR), Boston, MA, USA, 2015.
[https://doi.org/10.1109/CVPR.2015.7298878]
-
S. Ji, W. Xu, M. Yang, and K. Yu, “3d convolutional neural networks for human action recognition,” IEEE Transactions on Pattern Analysis and Machine Intelligence, vol. 35, no. 1, pp. 221-231, 2013.
[https://doi.org/10.1109/TPAMI.2012.59]
-
G. W. Taylor, R. Fergus, Y. LeCun, and C. Bregler, “Convolutional learning of spatio-temporal features,” European Conference on Computer Vision, pp. 140-153, 2010.
[https://doi.org/10.1007/978-3-642-15567-3_11]
-
D. Tran, L. Bourdev, R. Fergus, L. Torresani, and M. Paluri, “Learning spatiotemporal features with 3d convolutional networks,” 2015 IEEE International Conference on Computer Vision (ICCV), Santiago, Chile, 2015.
[https://doi.org/10.1109/ICCV.2015.510]
-
G. Varol, I. Laptev, and C. Schmid, “Long-term temporal convolutions for action recognition,” IEEE Transactions on Pattern Analysis and Machine Intelligence, 2017.
[https://doi.org/10.1109/TPAMI.2017.2712608]
-
A. Wedel, T. Pock, C. Zach, H. Bischof, and D. Cremer, “An improved algorithm for tv-l 1optical flow,” Statistical and Geometrical Approaches to Visual Motion Analysis, pp. 23-45. 2009.
[https://doi.org/10.1007/978-3-642-03061-1_2]
-
N. Crasto, P. Weinzaepfel, K. Alahari, and C. Schmid, “MARS: Motion-augmented RGB stream for action recognition,” 2019 IEEE/CVF Conference on Computer Vision and Pattern Recognition (CVPR), Long Beach, CA, US, 2019.
[https://doi.org/10.1109/CVPR.2019.00807]
2019 한밭대학교 정보통신공학과(학사)
2020~현재 한밭대학교 정보통신대학원(석사)
관심분야: Robotics, Vision Programing, Deep Learning