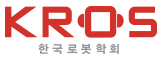
열화상 이미지 다중 채널 재매핑을 통한 단일 열화상 이미지 깊이 추정 향상
CopyrightⓒKROS
Abstract
Depth information used in SLAM and visual odometry is essential in robotics. Depth information often obtained from sensors or learned by networks. While learning-based methods have gained popularity, they are mostly limited to RGB images. However, the limitation of RGB images occurs in visually derailed environments. Thermal cameras are in the spotlight as a way to solve these problems. Unlike RGB images, thermal images reliably perceive the environment regardless of the illumination variance but show lacking contrast and texture. This low contrast in the thermal image prohibits an algorithm from effectively learning the underlying scene details. To tackle these challenges, we propose multi-channel remapping for contrast. Our method allows a learning-based depth prediction model to have an accurate depth prediction even in low light conditions. We validate the feasibility and show that our multi-channel remapping method outperforms the existing methods both visually and quantitatively over our dataset.
Keywords:
Thermal Camera, Depth Estimation1. 서 론
깊이 정보는 환경을 표현하는 매우 중요한 요소이다. 많은 연구자들이 이미지를 픽셀 별로 분류할 때나 연속된 이미지에서 회전 및 움직인 거리를 구할 때, 동시적 위치추적 및 지도작성(SLAM, Simultaneous Localization And Mapping), 물체 인식 등에 깊이 정보를 사용한다. 깊이 정보는 스테레오 카메라나 움직이는 카메라의 위치 변화를 추적하는데 이용되며 라이다(LiDAR, Light Detection And Ranging)등의 센서로부터 얻을 수 있다. RGB 스테레오 이미지에서 geometry를 기반으로 깊이를 추정하는 연구에서부터 단일 RGB 이미지에 딥 러닝을 기반으로 깊이 추정 연구까지 활발히 진행되고 있다. 하지만 RGB 이미지는 밤이나 동굴과 같이 빛이 적은 환경에서는 이미지에 물체 등이 나타나지 않기에 적합하지 않다.
열화상 이미지는 가시광선이 아닌 적외선으로부터 온 도를 얻는 센서이기 때문에 빛이 적은 환경에서도 문제 없이 작동한다. 하지만 열화상 이미지는 어두운 부분과 밝은 부분의 차이가 작고 질감이 낮다는 큰 단점이 있다. 이 문제를 풀기 위해 연구자들은 histogram equalization를 이용하거나 딥 러닝을 기반으로 열화상 이미지의 품질을 개선하려고 하였다. 하지만 이 과정에서 장면에 영향을 받아 같은 온도이지만 이미지 픽셀 값이 달라지게 되고, 이로 인해 스테레오 매칭이 안되는 등의 문제가 발생한다. 본 논문에서는 장면에 영향을 받지 않으면서 풍부한 질감, 어두운 부분과 밝은 부분의 차이를 극대화 하는 새로운 품질 개선 방법을 제안한다[Fig. 1].
![[Fig. 1] [Fig. 1]](/xml/33768/JKROS_2022_v17n3_314_f001.jpg)
The propose 3-channel remapping for a thermal image exaggerates the variation within a thermal image increasing interpretability. The 3-channel thermal remapping can express the structure that was not expressed in the raw thermal image such as ArUco[1] (first row)
딥 러닝을 이용할 때는 LiDAR나 스테레오 이미지의 시차를 이용해 라벨링하여 깊이를 학습시킨다. 스테레오 이미지에서 얻은 시차는 LiDAR보다 정확도가 낮고 절대 깊이를 알 수 없으나 이미지의 모든 픽셀에 대응하는 깊이를 알 수 있다. 반면 LiDAR로 얻은 깊이는 후처리 과정을 거치지 않는다면 단지 이미지의 10%정도에 해당하는 깊이 정보만을 얻을 수 있다. 본 논문에서는 두 센서가 갖는 단점들을 서로 보완할 수 있다고 생각하여 깊이 추정의 정확도를 높일 수 있는 LiDAR와 스테레오 시차를 이용한 손실 함수를 제시한다.
최종적으로, 아래의 항목들은 본 논문에서 제시하고자 하는 논점이다.
- • 열화상 이미지에서 질감을 높이고 많은 정보를 저장할 수 있는 N- channel thermal remapping function을 제안하여 단일 열화상 이미지 깊이 추정에 도움을 준다.
- • LiDAR에 얻은 정확하지만 적은 깊이 정보와 스테레오 이미지에서 얻은 덜 정확하지만 풍부한 깊이 정보를 이용하는 손실 함수를 제시한다.
- • 우리 데이터셋에서 다른 방법들보다 3.79% 개선된 성능을 보였다.
2. 선행 연구 조사
2.1 열화상 이미지 품질 개선
열화상 이미지는 빛이 적은 환경에서도 강건하나 질감이 부족하고 어두운 부분과 밝은 부분의 차이가 적으며 열화상 카메라에서 갖는 열로 인한 노이즈가 생긴다는 단점이 있다. 이를 해결하기 위해 Dulski[3]는 histogram equalization를 이용한 열화상 품질 개선 연구를 하였다. Tran[4]는 histogram equalization 방법에 입력 데이터를 기반으로 하는 bi-dimensional 함수를 만들어 분석하는 방법(BEMD, Bidimensional empirical mode decomposition)을 사용하였다. Histogram을 기반으로 한 방법들은 장면에 영향을 받아 같은 온도임에도 장면마다 다른 값을 갖게 되어 특징 매칭에 어려움을 준다. Agaian와 Roopaei[5]은 sigmoid 기반 비선형 함수로 이미지의 값을 바꾸어 이미지 품질을 향상시켰으나 온도 범위가 넓은 큰 실외 환경에서는 적합하지 않다. 최근에는 딥 러닝을 기반으로 열화상 이미지 품질을 개선하려는 방향으로 연구가 활발히 진행되고 있다. Choi[6]는 합성곱 신경망(CNN, Convolutional neural network)을 기반으로 열화상 이미지에 RGB 이미지를 학습시켜 이미지 품질을 개선하였으며 Lee[7]는 밝기 영역을 통합하여 열화상 이미지 품질을 개선하였다. 이러한 방법들은 학습에 열화상 이미지 이외에 RGB 이미지가 추가적으로 더 필요하기에 데이터 셋에 열화상 이미지만 존재한다면 학습을 시킬 수 없다는 큰 문제가 있다. 본 논문에서는 실외 환경에서 열화상 품질을 높이면서 장면에 영향을 받지 않는 열화상 재 매핑 함수를 제안한다.
2.2 깊이 추정
깊이 추정은 다양한 분야에 쓰이기에 오래전부터 많은 연구가 지속되어 왔다. Shah와 Aggarwal[8]은 스테레오 fish-eye 렌즈를, Rajagopalan[9]은 defocused 스테레오 쌍을 이용하여 깊이를 추정하였다. Liu[10]은 다시점 스테레오 이미지에서의 광학 흐름을 이용하여 다양한 스케일 레벨에서 변동된 깊이를 추정하는 다중 시작 스케일 프레임 워크를 제안했다. 많은 연구자들은 스테레오 이미지에서 geometry를 기반으로 깊이를 추정하였으나 특별한 센서가 필요하거나 스테레오 이미지에서만 깊이를 추정하였기에 단일 이미지에서의 깊이 추정은 여전히 해결하지 못하였다.
이후 단일 이미지에서 깊이 추정을 하기 위해 뉴럴 네트워크를 기반으로 연구가 많이 진행되었다. Eigen[11]는 깊이 추정을 위해 전체 이미지를 기반으로 대략적인 전역 예측을 수행하는 네트워크와 이 예측을 로컬로 수정하는 네트워크, 총 두개의 CNN을 제안하였고 Laina[12]은 깊이 지도에서의 분포를 Huber 손실을 이용하여 학습하였다. 또다른 방법으로 Ranftl[13]은 epipolar 조건을 이용해 시차 이미지를 만들어 셀프 라벨링을 하여 학습하는 방법도 제안하였다.
최근에는 열화상 이미지에서도 깊이 추정 연구가 활발히 진행되고 있다. Kim[14]는 스테레오 RGB 이미지의 시차를 계산하여 열화상 이미지에 학습시켜 깊이 추정을 하였다. Lu[15]는 왼쪽 스테레오 RGB 이미지를 전이시킨 열화상 이미지와 오른쪽에 위치한 열화상 카메라로부터 얻어진 이미지를 활용하여 깊이를 추정하였다. 기존 방법과 비교하면 우리의 방법은 별도의 RGB 이미지 및 RGB-열화상 카메라 calibration 매개변수 필요가 없다.
3. 연구 방법
3.1 열화상 이미지 품질 개선 방법
이번 논문의 전체적인 순서도는 [Fig. 2]와 같다. 열화상 이미지는 RGB 이미지보다 질감이 부족하고 어두운 부분과 밝은 부분의 차이가 작아 특징 추출이 어렵다. 이 문제를 해결하기 위해 본 논문에서는 새로운 열화상 이미지 품질 개선 방법을 제안한다. 열원을 제외한 물체는 일반적으로 전체 면적에 대한 온도 분포가 거의 균등하다. 그러나 물체 간의 온도 차는 햇빛, 열원과의 거리 등의 이유로 일정하지 않게 측정된다. 그래서 본 논문에서는 주기가 짧은 사인함수를 기반으로 한 새로운 열화상 재매핑 함수g(x)를 제안한다. 이 함수를 통해 온도 공간에서 값의 차이가 작아 식별이 불가하였지만, 픽셀 공간으로 매핑함으로써 값의 차이가 확연히 드러나는 것을 알 수 있다[Fig. 3].
(1) |
![[Fig. 2] [Fig. 2]](/xml/33768/JKROS_2022_v17n3_314_f002.jpg)
Overview. Our model consists of 3 steps. First (blue box), the stereo depth map (DS) is obtained by feeding the remapped 3-channel thermal images (IlTRI, IrTRI) through FrowNet[2]. Second (red box), multi-channel thermal images (IlNRI, IrNRI) are constructed. Third (green box), the predicted depth map (DP) is trained by feeding the remapped IlNRI, IrNRI, LiDAR depth map (DL), and DS through Depth network
![[Fig. 3] [Fig. 3]](/xml/33768/JKROS_2022_v17n3_314_f003.jpg)
The top image is a raw image, and the right figure is an image converted using. g(x). (a) means temperature difference, and (b) is pixel value difference
R은 주기를 정하기 위한 온도의 범위, T는 평행이동을 위한 온도이다.
3.2 단일 이미지에서의 깊이 예측
이 섹션에서는 단일 열화상 이미지에서 깊이 예측 방법을 설명한다. 본 논문에서는 LiDAR와 스테레오 열화상 이미지 데이터가 있다고 가정하고 센서들의 calibration 매개변수는 알고 있다고 가정하였다.
본 논문에서는 g(x)의 R 값을 변화 시켜 여러 개의 열화상 재매핑 이미지를 쌓아 N-채널 열화상 재매핑 이미지를 만들었다. [Fig. 4]를 통해 같은 영역이지만 R 값에 따라 다르게 보이는 것을 확인 할 수 있다. 또한, 여러 개의 열화상 재매핑 이미지를 쌓으면 많은 정보를 담을 수 있다는 것을 확인하였다.
![[Fig. 4] [Fig. 4]](/xml/33768/JKROS_2022_v17n3_314_f004.jpg)
The example of 3-channel thermal remapping image. The second row represents three 1-channel thermal remapping images. The first row shows visibility difference between three thermal remapping images
학습에 앞서, 일반적인 영역에 비하여 하늘은 거리가 매우 멀리 떨어져 있기 때문에 깊이를 학습하는 데 방해가 된다. 그러나 RGB 이미지와는 다르게 열화상 이미지에서 하늘은 다른 영역보다 온도가 낮아 복잡한 알고리즘 없이 쉽게 제거할 수 있다. 이 특성을 g(x)에 적용하여 식 (2)을 만들었다[Fig. 5].
(2) |
이때 Ts는 20°C로 사용하였다.
본 논문에서는 4개의 손실 함수를 사용하여 깊이를 학습하였다.
3.2.2.1 스테레오 손실 함수(Lstereo)
스테레오 이미지에서 시차를 구하기 위해 필요로 하는 것은 intrinsic calibration 매개변수와 extrinsic calibration 매개변수이다. 하지만 열화상 이미지는 RGB 이미지와 성질이 다르기 때문에 RGB 이미지에서 intrinsic calibration 매개변수를 구하는 방법[16]은 정확도가 떨어진다. Ranftl[13]는 calibration 매개변수를 모를 때 optical flow를 시차 대신 사용하여 깊이 추정에 성공적인 결과를 보였다. 이를 착안하여 본 논문에서는 optical flow를 시차 대신 ground truth inverse 깊이 맵 DS로 사용하였다. DS와 추정한 inverse 깊이 맵 DP는 밑의 식 (3)으로 정규화 한 후 둘의 L1-norm을 손실 한수 Lstereo로 사용하였다.
이때 d는 inverse 깊이이다.
(3) |
3.2.2.2 LiDAR 손실 함수(LLiDAR)
LLiDAR는 LiDAR에서 inverse 깊이 맵 DL과 DP사이의 L2-norm을 사용하였다. 이 과정에서 DL와 DP의 스케일이 다르기 때문에 식 (4)를 통해 LiDAR 기준의 스케일로 맞추었다.
(4) |
3.2.2.3 Photometric 에러 손실 함수(LP)
LP는 [17-20]에서 photometric reprojection 에러를 사용하여 깊이를 학습하였을 때 높은 성능을 보인 것을 바탕으로 다음과 같이 정의하였다.
(5) |
이때 K는 intrinsic 변수, Tl→r은 extrinsic 변수, TlTRI는 왼쪽 이미지, TrTRI는 오른쪽 이미지, eP는 왼쪽 이미지를 오른쪽 이미지로 reprojection 시켰을 때 같은 픽셀에서 세기 차이를 뜻한다. 본 논문에서는 eP를 L1-norm과 SSIM (structural similarity index metric)를 함께 사용하여 정의하였다[21]. 이때 α는 0.85로 사용하였다.
(6) |
3.2.2.4 Gradient 손실 함수(Lgrad)
본 논문에서는 이미지에서 모서리와 같은 불연속 부분을 학습시키기 위해 multi-scale invariant gradient 매칭 손실 함수 Lgrad를 사용하였다[13,22].
이때 scale(N)은 4를 사용하였고, 이다. 최종 손실 함수는 다음과 같다.
4. 연구 결과
이 절에서는 깊이 추정의 성능과 다중 채널 열 재매핑 이미지의 효과를 평가한다.
4.1 데이터 셋
우리 데이터 셋은 LiDAR, 스테레오 열화상 이미지 및 calibration 매개 변수를 제공하고 다양한 시간대의 많은 시퀀스를 취득하여 연구를 진행하였다. 학습에 하기에 앞서 본 논문에서는 2가지 과정을 거쳐 학습에 쓰일 데이터를 필터링하였다. 열화상 카메라에는 카메라의 온도로 인한 노이즈를 보정하기 위해 비균일 보정(NUC, Non-uniformity Correction)기능이 탑재되어 있다. 이로 인해 열화상 카메라가 데이터 취득 도중 꺼지는 경우가 발생한다. 노이즈를 제거한다는 장점이 있지만 NUC를 임의로 제어할 수 없어 모든 열화상 스테레오 이미지가 쌍을 이루고 있지 않다. 학습에는 열화상 스테레오 쌍이 필요하므로 타임 스탬프를 기반으로 쌍을 이루지 않는 데이터를 필터링하였다. 다음은 left-right consistency가 0.7보다 큰 픽셀이 전체의 30% 이상 있는 경우 학습에 적합하지 않다고 판단하여 제외하였다. 총 50,724개의 이미지가 학습에 사용되었고 8,122개의 이미지로 검증하였다.
본 논문에서는 깊이 추정을 위한 다중 채널 열화상 재매핑 이미지를 만들기 위해 식 (2)에서 Ri를 4°C부터 0.3°C씩 증가시켜 이미지들을 쌓았다. 24G 메모리가 있는 한 개의 GTX-3090 GPU를 사용하여 75시간동안 50 epoch의 학습을 진행하였다. 러닝 레이트는 처음 7개의 epoch동안 10-4로 시작하였고 나머지 epoch에서는 10-5로 감소 시켜 사용하였다.
4.2 단일 깊이 추정
본 논문에서는 정량 평가를 위해 예측한 깊이를 식 (4)를 통해 스케일 보정 후 LiDAR에서 얻은 절대 깊이와 비교하여 평가하였다. 우리의 모델과 최첨단(SOTA, State Of The Art) 모델의 정량 평가는 절대 상대 차이(AbsRel, Absolute Relative difference), 상대 차이 제곱(SqRel, Squared Relative difference), 제곱 평균 제곱근 에러(RMSE, Root Mean Square Error), RMSE log, δ < 1.25, δ < 1.252, δ < 1.253 7가지 평가지표로 평가한다.
는 스케일을 보정한 예측 깊이, 은 LiDAR의 절대 깊이이다.
[Table 1]을 보면 우리의 모델이 Monodepth2[19], BTS[23], MiDAS[13] 모델보다 7가지 평가 지표에서 앞서는 것을 알 수 있다. 또한, [Fig. 6]에서는 다른 SOTA모델들은 하늘과 땅의 깊이를 반대로 예측하거나 차의 보닛을 전혀 보지 못하는 등 깊이 추정에 실패하는 것을 알 수 있다.
4.3 열화상 재 매핑 이미지 효과
본 소단락에서는 다중 채널 열화상 재매핑 이미지가 깊이 추정에 미치는 영향을 검증한다. 비교를 위해 본 논문에서는 열화상 이미지를 개선시키지 않은 Raw 이미지, 1, 2, 3, 4, 5, 10-채널 이미지들로 깊이 추정을 학습하여 비교하였다. 모든 평가는 4.2에 제시된 7가지 평가지표를 사용하였다. Raw 데이터와 5-채널 열화상 재매핑 이미지로 깊이를 이용한 깊이 추정 결과는 [Table 1]에 나타나있다. 다중 채널 열화상 재매핑 이미지 모델이 raw 데이터 모델보다 RMSE log를 제외한 다른 평가지표에서 우수한 성능을 보여준다.
채널수에 따른 결과는 [Fig. 7]과 [Fig. 8], [Table 2]에서 확인할 수 있다. [Fig. 7]은 채널수가 1부터 5까지 증가할 때 RMSE 값의 점차 줄어들고 그 이후부터 다시 증가하는 것을 보여준다. 또한, [Table 2]에서는 RMSE뿐만 아니라 다른 6가지 평가지표에서도 채널 수가 적을 때보다 많을 때 개선된 결과가 나온 것을 보여준다. [Fig. 8]는 채널 수에 따른 깊이 예측 결과이다. 특히, 1, 2, 3 열에서 1, 2채널일때와 3, 4, 5, 10 채널 일 때 극명한 차이가 나타나는 것을 알 수 있다.
![[Fig. 7] [Fig. 7]](/xml/33768/JKROS_2022_v17n3_314_f007.jpg)
This graph shows that when the number of channels increases from 1 to 5, the RMSE decreases, and when the number of channels increases to 10, the RMSE increases
![[Fig. 8] [Fig. 8]](/xml/33768/JKROS_2022_v17n3_314_f008.jpg)
The qualirative inverse depth map evaluation according to changing the number of channels of thermal remapping image
5. 결 론
본 연구에서는 짧은 주기 함수를 이용한 다중 채널 열화상 재매핑 이미지 기법을 도입하였다. 이 기법은 열화상 이미지에서 질감을 높이고 많은 정보를 담는 등의 품질을 개선하였다. 또한, 로보공학에서 매우 중요한 깊이 정보를 얻는 방법에 다중 채널 열화상 재매핑 이미지 기법을 적용하였다. 실험은 다중 채널 열화상 재매핑 이미지 기법이 깊이 추정에 끼치는 영향과 채널 수가 깊이 추정에 끼치는 영향을 확인하였다. 3채널 이상의 다중 채널 열화상 재매핑 이미지로 학습한 모델은 Raw 이미지나 1, 2채널 이미지로 학습한 모델보다 유의미한 차이를 보였으며 기존의 SOTA 모델들보다도 깊이를 잘 예측하였다. 이번 논문을 통해 다중 채널 열화상 재매핑 이미지가 열화상 카메라의 단점을 보완할 수 있고 빛이 적은 환경에서 열화상 이미지를 RGB 이미지처럼 사용 할 수 있을 것이라는 가능성을 보여주었다.
Acknowledgments
This work is supported by a grant (22TSRD-C151228-04) from Urban Declining Area Regenerative Capacity-Enhancing Technology Research Program funded by Ministry of Land, Infrastructure and Transport of Korean government
References
-
F. J. Romero-Ramirez, R. Muoz-Salinas, and R. MedinaCarnicer, “Speeded up detection of squared fiducial markers,” Image and Vision Computing, vol. 76, August, 2018.
[https://doi.org/10.1016/j.imavis.2018.05.004]
-
E. Ilg, N. Mayer, T, Saikia, M. Keuper, A. Dosovitskiy, and T. Brox, “Flownet 2.0: Evolution of optical flow estimation with deep networks,” 2017 IEEE Conference on Computer Vision and Pattern Recognition (CVPR), Honolulu, HI, USA, 2017.
[https://doi.org/10.1109/CVPR.2017.179]
-
R. Dulski, P. Powalisz, M. Kastek, and P. Trzaskawka, “Enhancing image quality produced by IR cameras,” Electro-Optical and Infrared Systems: Technology and Applications VII, 2010.
[https://doi.org/10.1117/12.864979]
-
V. T. Tran, B.-S. Yang, F. Gu, and A. Ball, “Thermal image enhancement using bi-dimensional empirical mode decomposition in combination with relevance vector machine for rotating machinery fault diagnosis,” Mechanical Systems and Signal Processing, vol. 38, no. 2, pp. 601-614, July, 2013.
[https://doi.org/10.1016/j.ymssp.2013.02.001]
-
S. Agaian and M. Roopaei, “Novel infrared and thermal image enhancement algorithms,” Mobile Multimedia/Image Processing, Security, and Applications 2013, 2013.
[https://doi.org/10.1117/12.2016040]
-
Y. Choi, N. Kim, S. Hwang, and I. S. Kweon, “Thermal image enhancement using convolutional neural network,” 2016 IEEE/RSJ International Conference on Intelligent Robots and Systems (IROS), Daejeon, South Korea, 2016.
[https://doi.org/10.1109/IROS.2016.7759059]
-
K. Lee, J. Lee, J. Lee, S. Hwang, and S. Lee, “Brightness based convolutional neural network for thermal image enhancement,” IEEE Access, vol. 5, 2017.
[https://doi.org/10.1109/ACCESS.2017.2769687]
-
S. Shah and J. K. Aggarwal, “Depth estimation using stereo fish-eye lenses,” 1st International Conference on Image Processing, Austin, TX, USA, 1994.
[https://doi.org/10.1109/ICIP.1994.413669.]
-
A. N. Rajagopalan, S. Chaudhuri, and Uma Mudenagudi, “Depth estimation and image restoration using defocused stereo pairs,” IEEE Transactions on Pattern Analysis and Machine Intelligence, vol. 26, no. 11, Nov., 2004.
[https://doi.org/10.1109/TPAMI.2004.102]
-
Y. Liu, X. Cao, Q. Dai, and W. Xu, “Continuous depth estimation for multi-view stereo,” 2009 IEEE Conference on Computer Vision and Pattern Recognition, Miami, FL, USA, 2009.
[https://doi.org/10.1109/CVPR.2009.5206712]
- D. Eigen, C. Puhrsch, and R. Fergus, “Depth map prediction from a single image using a multi-scale deep network,” arXiv:1406.2283, 2014.
-
I. Laina, C. Rupprecht, V. Belagiannis, F. Tombari, and N. Navab, “Deeper depth prediction with fully convolutional residual networks,” 2016 Fourth international conference on 3D vision (3DV), Stanford, CA, USA, 2016.
[https://doi.org/10.1109/3DV.2016.32]
-
R. Ranftl, K. Lasinger, D. Hafner, K. Schindler, and V. Koltun, “Towards robust monocular depth estimation: Mixing datasets for zero-shot cross-dataset transfer,” IEEE Transactions on Pattern Analysis and Machine Intelligence, vol. 44, no. 33, March, 2020.
[https://doi.org/10.1109/TPAMI.2020.3019967]
-
N. Kim, Y. Choi, S. Hwang, and I. S. Kweon, “Multispectral Transfer Network: Unsupervised Depth Estimation for All-Day Vision,” Thirty-Second AAAI Conference on Artificial Intelligence, vol. 32, no. 1, 2018, [Online], https://ojs.aaai.org/index.php/AAAI/article/view/12297, .
[https://doi.org/10.1609/aaai.v32i1.12297]
-
Y. Lu and G. Lu, “An alternative of lidar in nighttime: Unsupervised depth estimation based on single thermal image,” 2021 IEEE Winter Conference on Applications of Computer Vision (WACV), Waikoloa, HI, USA, 2021.
[https://doi.org/10.1109/WACV48630.2021.00388.]
-
Z. Zhang, “A flexible new technique for camera calibration,” IEEE Transactions on Pattern Analysis and Machine Intelligence, vol. 22, no. 11, Nov., 2000.
[https://doi.org/10.1109/34.888718]
-
T. Zhou, M. Brown, N. Snavely, and D. G. Lowe, “Unsupervised learning of depth and ego-motion from video,” arXiv:1704.07813, 2017.
[https://doi.org/10.1109/CVPR.2017.700]
-
R. Garg, B. G. V. Kumar, G. Carneiro, and I. Reid, “Unsupervised cnn for single view depth estimation: Geometry to the rescue,” European Conference on Computer Vision, 2016.
[https://doi.org/10.1007/978-3-319-46484-8_45]
-
C. Godard, O. M. Aodha, M. Firman, and G. Brostow, “Digging into self-supervised monocular depth estimation,” 2019 IEEE/CVF International Conference on Computer Vision (ICCV), Seoul, Korea (South), 2019.
[https://doi.org/10.1109/ICCV.2019.00393]
-
C. Godard, O. M. Aodha, and G. J. Brostow, “Unsupervised monocular depth estimation with left-right consistency,” 2017 IEEE Conference on Computer Vision and Pattern Recognition (CVPR), Honolulu, HI, USA, 2017.
[https://doi.org/10.1109/CVPR.2017.699]
-
Z. Wang, A. C. Bovik, H. R. Sheikh, and E. P. Simoncelli, “Image quality assessment: from error visibility to structural similarity,” IEEE Transactions on Image Processing, vol. 13, no. 4, Apr., 2004.
[https://doi.org/10.1109/TIP.2003.819861]
-
Z. Li and N. Snavely, “Megadepth: Learning single-view depth prediction from internet photos,” 2018 IEEE/CVF Conference on Computer Vision and Pattern Recognition, Salt Lake City, UT, USA, 2018.
[https://doi.org/10.1109/CVPR.2018.00218]
- J. H. Lee, M.-K. Han, D. W. Ko, and I. Suh, “From big to small: Multi-scale local planar guidance for monocular depth estimation,” arXiv:1907.10326, 2019.
2020 KAIST 수리과학부(공학사)
2021~현재 KAIST 건설 및 환경공학과 석사과정
관심분야: Computer Vision, SLAM
2017 광운대학교 로봇학부(공학사)
2020 KAIST 로봇공학제(공학사)
2020~현재 KAIST 로봇공학제 박사과정
관심분야: SLAM, Computer Vision
2005 서울대학교 기계항공공학과(공학사)
2007 서울대학교 기계항공공학전공(공학석사)
2007 서울대학교 기계항공공학전공(공학석사)
2014~현재 한국과학기술원 건설 및 환경공학과 부교수
관심분야: 영상기반 SLAM