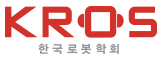
실내 환경에서의 레이저 반사도를 고려한 라이다 기반 지도 작성
CopyrightⓒKROS
Abstract
Light detection and ranging (LiDAR) sensors have been most widely used in terrestrial robotic applications because they can provide dense and precise measurements of the surrounding environments. However, the reliability of LiDAR measurements can considerably vary due to the different reflectivities of laser beams to the reflecting surface materials. This study presents a robust LiDAR-based mapping method for the varying laser reflectivities in indoor environments using the framework of simultaneous localization and mapping (SLAM). The proposed method can minimize the performance degradations in the SLAM accuracy by checking and discarding potentially unreliable LiDAR measurements in the SLAM front-end process. The gaps in point-cloud maps created by the proposed approach are filled by a Gaussian process regression method. Experimental results with a mobile robot platform in an indoor environment are presented to validate the effectiveness of the proposed methodology.
Keywords:
LiDAR SLAM, Laser Reflectivity, Gaussian Process Regression1. 서 론
로봇공학 및 센싱 기술이 지속해서 발전함에 따라, 라이다(LiDAR: Light detection and ranging)는 정밀 구조 측정, 객체 탐지 및 인식, 동시적 위치추정 및 지도작성(SLAM: Simultaneous localization and mapping) 등과 같은 지상 환경에서의 로봇 응용 분야에 가장 널리 사용되어 온 센서 중 하나이다. 라이다 센서는 기본적으로 방향각 분해능에 따라 개별 레이저 빔(Laser beam)을 출력하고, 대상 표면에 반사되어 돌아온 레이저 빔의 비행시간(Time-of-flight)과 여러 물리적 속성들을 고려하여 계산된 거리 계측치를 제공한다. 그러나, 유리나 거울과 같이 실내 환경에서 흔히 볼 수 있는 투명 표면(Transparent surface)이나 정반사 표면(Specular reflective surface)에서는 레이저 빔의 입사각에 따라 반사 신호 강도(Intensity)가 크게 달라질 수 있으며, 이러한 상황에서 제공되는 라이다 계측치의 신뢰도는 급격히 저하될 수 있다. 표면 반사도 차이로 인한 라이다 거리 계측치의 신뢰도 변화 예시를 [Fig. 1]에 보인다.
![[Fig. 1] [Fig. 1]](/xml/36674/JKROS_2023_v18n2_135_f001.jpg)
An example of varying reliability of LiDAR measurements due to the different reflectivities of laser beams to the reflecting surface materials: (a) LiDAR measurements obtained by a typical concrete wall. (b) LiDAR measurements obtained by a specular reflective surface (i.e., a mirror)
본 연구에서는 실내 환경에 존재하는 여러 표면에 대해 레이저 반사도(Reflectivity)가 일정하지 않은 상황에서도 강건하게 동작할 수 있는 라이다 기반 지도작성(Mapping) 방법을 제안한다. 사전 정보가 제공되지 않은 실내 환경에서 비컨(Beacon) 기반의 절대 위치 계측 없이 이동로봇 플랫폼을 이용한 정밀 지도를 생성하기 위해, 제안된 방법은 라이다 SLAM 알고리즘을 기반으로 한다.
표면 반사도가 일정하지 않은 실내 환경에서 라이다 SLAM 알고리즘을 적용한 몇몇 연구 사례들이 존재한다. Yang[1]은 실내 환경에서 라이다 기반 지도 작성 수행 시 거울 표면 반사에 의해 점군(Point cloud) 지도의 일관성이 저하되는 문제를 해결하고자, 라이다 계측치만을 사용하여 거울을 검출하고 이를 지속적으로 추적하는 베이지안 추정(Bayesian estimation) 방법을 제안하였다. Wang[2]은 실내 환경에 존재하는 여러 표면에 대한 레이저 빔의 고유 반사도 특성을 고려하여 유리 표면을 검출하고, 이를 Rao-Blackwellized 입자필터(RBPF: Rao-Black wellized particle filter) 기반의 Gmapping[3] 알고리즘과 결합하여 지도 작성 결과의 성능 개선도를 보였다.
본 연구에서는 실내 환경에 존재하는 여러 표면 재질에 대하여 실험적으로 구축된 레이저 빔의 반사도 데이터베이스(DB: Database)를 이용하여, 신뢰도가 낮을 것으로 예측되는 라이다 계측치들을 SLAM 전단부(Front-end)에서 미리 배제하여 위치 추정 및 지도 작성 성능 저하를 최소화하고 해당 과정에서 발생하는 지도 상의 빈 영역을 최대한 정밀히 채우는 것에 초점을 둔다. 이를 위하여 스캔 필터링 과정과 후처리 회귀(Regression) 기법이 적용된다. 본 연구에서 제안된 실내 지도 작성 방법의 전체 흐름선도를 [Fig. 2]에 보인다. 이어지는 2장에서는 본 연구에서 제안된 방법에 대한 전반적인 내용을 소개하고, 제안된 알고리즘의 유용성을 검증하기 위한 실험 결과들이 3장에서 제시된다. 4장에서는 본 연구의 결론이 기술된다.
2. 제안된 라이다 기반 지도 작성 방법
2.1 레이저 반사도를 고려한 스캔 필터링 과정
본 절에서는 신뢰도 낮을 것으로 예측되는 라이다 계측치를 사전에 배제하기 위한 스캔 필터링(Scan filtering) 과정에 대해 소개한다. 일반적인 라이다 센서들은 각 레이저 빔이 반사된 표면에 대한 상대 방향각 및 거리와 더불어 식 (1)과 같이 정의되는 반사 강도, I 를 계측하여 제공한다[4].
(1) |
여기서, η는 라이다 센서의 고유한 보정(Calibration) 상수를, ρ는 표면 반사도를 나타낸다. α와 R은 각각 레이저 빔의 입사각과 물체와의 거리를 의미한다. [Fig. 3]은 실내 환경에서 관측 가능한 여러 물체 표면들을 같은 거리에 두고 실험적으로 구축된 레이저 반사도 DB의 한 예를 보인다. 레이저 빔이 근사적으로 수직 입사되는 경우들 외에는 유리와 같은 투명 표면이나 거울과 같은 정반사 표면에서 확연히 낮은 반사 강도 값이 계측됨을 확인할 수 있다. 본 연구에서 제안된 스캔 필터링 과정은 원본 라이다 계측치 중 일정 수준 이하의 반사 강도 값을 가지는 라이다 점군 데이터와 인접 점군 데이터를 임계 처리(Thresholding)를 통해 제거하여 SLAM 추정기로 입력한다. 본 연구에서는 실험적으로 구축된 반사도 DB 정보를 참조하여 반사 강도 임계 처리 값을 20으로 설정한다. 식 (1)에 따르면 대상 표면과의 거리가 멀수록 반사 강도의 값이 크게 감소하므로, 정상적인 라이다 계측치도 스캔 필터에 의해 제거될 수가 있는데, 이동로봇 플랫폼을 이용하는 방식의 특성상 지도 작성을 수행하는 대상 영역 전체를 1회 이상 탐사하면 정상적인 라이다 계측 신뢰도를 제공하는 표면 영역은 근접 거리에서 다시 복원될 수 있다.
2.2 가우시안 프로세스 회귀 적용
스캔 필터링 과정을 통해 야기된 지도상의 빈틈을 특정 수학적 모델이나 기하학적 원형(Geometric primitives)의 가정 없이 최대한 채우기 위해, 비모수(Nonparametric) 회귀 기법인 가우시안 프로세스 회귀(GPR: Gaussian process regression)[5,6]를 사용한다. GPR을 위한 데이터들은 추정될 회귀 함수 모델에 대하여 일대일 대응 구조를 가지는 것이 바람직하나, 2차원 직교 좌표계 공간에 분포된 실내 점군 지도 위치 데이터들은 대부분 일대다 대응 구조를 가지게 된다. 본 연구에서는 이러한 문제를 해결하기 위해 SLAM으로 생성된 전체 지도 영역을 부분 지도(Submap)으로 분할하고, 각 국소 영역에 포함되는 점군 데이터들을 라이다 센서 좌표계 중심의 극 좌표계로 투영하여 GPR을 적용한다. k번째 부분 지도에서의 GPR을 위한 훈련 데이터셋(Training dataset) Dk는 식 (2)과 같이 구성된다.
(2) |
여기서, nk는 k번째 부분 지도에 존재하는 점군 데이터의 수를 의미한다. Xi와 Yi는 훈련 데이터 입력과 출력으로, 각각 라이다 좌표계와 각 계측점 간 상대 방향각(Bearing), βi 및 거리(Range), ρi로 정의된다. 유사하게, 시험 데이터셋(Test dataset)의 입력과 출력은 각각 스캔 필터로 걸러진 점군 데이터의 상대 방향각과 거리로 구성된다. 입력 데이터 각각의 분산값은 본 연구에서 사용된 라이다 센서(Velodyne VLP-16)의 고정밀 성능과 점군의 공간 분포 특성 위주로 회귀를 수행하고자 하는 목적에 따라, 동일한 미소값 0.001로 적용된다.
GPR 결과의 성능은 커널 함수(Kernel function)의 선정에 따라 크게 좌우된다. 본 연구에서는 Squared exponential, Exponential, Matern, Rational quadratic 커널 함수들을 실험적으로 적용한 결과, 다른 커널 함수 대비 실내 지도 점군 데이터에 대해 가장 우수한 회귀 성능을 보이는 식 (3)의 Exponential 커널 함수를 채택하여 적용한다.
(3) |
여기서, 과 LE는 하이퍼파라미터(Hyperparameter)로서 각각 Exponential 커널 함수의 분산 및 길이를 나타낸다. 본 연구에서는 데이터 후처리 단계에서 GPR을 적용하기 때문에 기본적으로 하이퍼파라미터값을 실험적으로 조정하여 결정한다. 는 GPR의 입력인 방향각 데이터의 분산값을 기준으로 미세 조정되고, LE는 3.3으로 설정된다.
[Fig. 4]에서 실내 점군 지도에 존재하는 빈 공간을 채우기 위해 Exponential 커널을 적용한 결과와 대표 비교군으로서 GPR에 가장 일반적으로 사용되는 Squared exponential 커널이 적용된 결과를 비교한다. 공간상에 간헐적으로 분포된 학습 데이터에 대하여 Exponential 커널을 적용한 경우 과추정(Overestimation) 정도가 크게 감소됨을 확인할 수 있다. Exponential 커널이 적용된 GPR이 보다 넓고 복잡한 구조의 실내 환경에 적용될 경우, 학습 데이터의 밀도가 너무 희소(Sparse)하거나 학습 데이터의 전반적인 분포 경향 대비 복잡한 국소 영역에서는 GPR로 추정된 점군 데이터 중 일부 데이터의 신뢰도가 하락할 수 있다. 본 연구에서는 실제적으로 지도 작성 결과의 일관성을 유지하는 데 초점을 두고자, 기존에 등록된 주변 점군 분포와의 거리와 분할된 부분 지도상 이동로봇 위치와의 거리를 고려하여 신뢰도가 낮다고 판단되는 GPR 추정 결과 점군 데이터들은 지도 작성에 사용하지 않는 방식을 적용한다.
3. 실험 결과
제안된 방법의 타당성과 실제적 유용성을 검증하기 위해 이동로봇을 이용한 실내 환경에서의 실험 결과가 제시된다. 실험에 사용된 [Fig. 5(a)]의 이동로봇 플랫폼은 바퀴 엔코더(Wheel encoder)와 AHRS (Attitude heading reference system) 센서로부터 각각 선속도와 각속도 오도메트리(Odometry) 정보를 계측하고, 상부에 장착된 Velodyne VLP-16 라이다 센서를 통해 주변 환경에 대한 점군 데이터를 계측한다. 실험은 [Fig. 5]에 보인 바와 같이 유리문 등의 큰 투명 표면과 거울 등의 정반사 표면이 다수 존재하는 계명대학교 공학 1호관 1층(Site A)과 공학 7호관 1층(Site B)에서 수행되었으며, 기본적인 SLAM 정확도 성능을 확보하기 위해 루프 폐쇄(Loop-closure)가 발생하도록 각 장소의 복도 전체를 주행하였다. 여기서, 제안된 방법의 유효성을 보이기 위한 베이스라인(Baseline) 라이다 SLAM 방법으로 Cartographer[7] 알고리즘을 선정 및 적용하였다.
![[Fig. 5] [Fig. 5]](/xml/36674/JKROS_2023_v18n2_135_f005.jpg)
Experimental mobile robot platform (Clearpath Husky UGV) in (a) Engineering Building No. 1 (Site A) and (b) Engineering Building No. 7 (Site B)
두 장소에서의 실험 데이터셋을 이용한 지도 작성 결과들을 [Fig. 6]과 [Fig. 7]에 보인다. 제안된 방법의 적용 없이 Cartog rapher SLAM 알고리즘만 적용한 결과들은 모두 투명 표면과 정반사 표면 근처에서 라이다 기반 루프 폐쇄 성능이 저하되어 실제 건물 구조도(Structural drawing) 대비 왜곡된 지도를 도출한다. 본 연구에서 제안된 스캔 필터링 과정이 적용된 경우에서는 루프 폐쇄의 성능 저하를 초래하는 부정확한 라이다 계측치들을 사전에 제거되어 SLAM의 추정 성능이 기본적으로 유지되나, 생성된 결과 지도상에 다수의 빈틈을 야기한다. 이러한 현상을 개선하기 위해 각 결과들에 제안된 GPR을 적용한 경우 빈틈이 채워진 지도를 도출할 수 있다. 제안된 방법의 성능 개선도를 정량적으로 평가하기 위한 지표로서 구조도를 기준(Reference)으로 한 지도 중첩률(Map overlap ratio)과 Roy에 의해 제안된 기하 편차 지표(Geometric deviation metric)[8] 기반의 지도 작성 오차를 사용한다. 와 를 각각 실제 건물 구조도 내의 기준 지도 점과 추정된 지도 점으로 정의할 때, 지도 중첩률은 점 개수 대비 점 개수의 백분율 비(Percent ratio)로 정의된다. 지도 작성 오차 ϵg은 식 (4)와 같이 정의된다.
![[Fig. 6] [Fig. 6]](/xml/36674/JKROS_2023_v18n2_135_f006.jpg)
Comparison between the estimated robot trajectory (blue lines) and pointcloud maps (red points) using the Site A dataset: (a) Structural drawing of the Site A which is considered as the reference data. The blue rectangles indicate the transparent and specular reflective surfaces in the Site A. The yellow circled numbers denote the waypoints designed for this experiment. (b) Result of the baseline Cartographer SLAM. (c) Result of the Cartographer SLAM with the scan filter considering laser reflectivity. (d) Result of applying the GPR to the result of (c). The actual structural drawing of Site A is used as the reference data for performance comparison
![[Fig. 7] [Fig. 7]](/xml/36674/JKROS_2023_v18n2_135_f007.jpg)
Comparison between the estimated robot trajectory (blue lines) and pointcloud maps (red points) using the Site B dataset: (a) Structural drawing of the Site B which is considered as the reference data. The blue rectangles indicate the transparent and specular reflective surfaces in the Site B. The yellow circled numbers denote the waypoints designed for this experiment. (b) Result of the baseline Cartographer SLAM. (c) Result of the Cartographer SLAM with the scan filter considering laser reflectivity. (d) Result of applying the GPR to the result of (c). The actual structural drawing of Site B is used as the reference data for performance comparison
(4) |
여기서,
식 (4)의 지도 작성 오차 지표 정의에 따라, 추정 성능 자체가 크게 저하되거나 지도에 빈틈이 많을수록 상대적으로 멀리 위치한 추정 지도 점을 선택하게 되어 자연히 큰 지도 작성 오차를 도출하게 된다. 각 실험의 정량적 성능 평가 결과를 정리한 [Table 1]과 [Table 2]을 통해 제안된 방법의 결과가 지도 중첩률 및 지도 작성 오차 지표에서 모두 가장 우수한 성능을 보임을 확인할 수 있다.
4. 결 론
본 논문에서는 실내 환경에서 신뢰도가 낮을 것으로 예측되는 라이다 계측치들을 미리 배제하는 스캔 필터링 과정을 도입함으로써, 보다 강건하게 동작할 수 있는 라이다 SLAM 기반 실내 지도 작성 방법을 제안하였다. 특히, 지도 형상 모델에 대한 가정 없이 스캔 필터링으로 야기된 지도상의 빈틈을 최대한 채우기 위해 후처리 GPR 과정을 도입하였다. 제안된 방법의 유효성 및 실제적 적용 가능성은 투명 표면과 정반사 표면 등이 존재하는 다른 두 실내 환경에서의 실험을 통해 검증되었다. 본 연구에서 제안된 방법은 투명 및 정반사 표면 등이 존재하는 건물의 실내 구조도를 새로 구축하거나, 기존 구조도와의 비교 및 결함 검출을 위한 목적으로 활용될 수 있다.
References
-
S.-W. Yang and C.-C. Wang, “On Solving Mirror Reflection in LIDAR Sensing,” IEEE/ASME Transactions on Mechatronics, vol. 16, no. 2, pp. 255-265, Apr., 2011.
[https://doi.org/10.1109/TMECH.2010.2040113]
-
X. Wang and J. Wang, “Detecting glass in simultaneous localisation and mapping,” Robotics and Autonomous Systems, vol. 88, pp. 97-103, Feb., 2017.
[https://doi.org/10.1016/j.robot.2016.11.003]
-
G. Grisetti, C. Stachniss, and W. Burgard, “Improving Grid-based SLAM with Rao-Blackwellized Particle Filters by Adaptive Proposals and Selective Resampling,” IEEE International Conference on Robotics and Automation (ICRA), Barcelona, Spain, 2005.
[https://doi.org/10.1109/ROBOT.2005.1570477]
-
H. Wang, C. Wang, and L. Xie, “Intensity-SLAM: Intensity Assisted Localization and Mapping for Large Scale Environment,” IEEE Robotics and Automation Letters, vol. 6, no. 2, pp. 1715-1721, Apr., 2021.
[https://doi.org/10.1109/LRA.2021.3059567]
-
C. E. Rasmussen and C. K. I. Williams, Gaussian Processes for Machine Learning, The MIT Press, 2005.
[https://doi.org/10.7551/mitpress/3206.001.0001]
-
S. Hong and J. Kim, “Three-dimensional visual mapping of underwater ship hull surface using piecewise-planar SLAM,” International Journal of Control, Automation and Systems, vol. 18, no. 3, pp. 564-574, 2020.
[https://doi.org/10.1007/s12555-019-0646-8]
-
W. Hess, D. Kohler, H. Rapp, and D. Andor, “Real-time loop closure in 2D LIDAR SLAM,” IEEE International Conference on Robotics and Automation (ICRA), Stockholm, Sweden, 2016.
[https://doi.org/10.1109/ICRA.2016.7487258]
-
M. Roy, S. Foufou, and F. Truchetet, “Mesh comparison using attribute deviation metric,” International Journal of Image and Graphics, vol. 4, no. 1, pp. 127-140, 2004.
[https://doi.org/10.1142/S0219467804001324]
2023 계명대학교 로봇공학(학사)
2023~현재 계명대학교 로봇시스템공학과 석사과정
관심분야: 로보틱스, SLAM, 자율 주행
2005 충남대학교 메카트로닉스공학(학사)
2007 충남대학교 메카트로닉스공학(석사)
2016 KAIST 기계공학(박사)
2016~현재 선박해양플랜트연구소(KRISO) 선임연구원
관심분야: 해양로봇, 지능항법 및 센서융합
2012 금오공과대학교 전자공학(학사)
2014 KAIST 로봇공학(석사)
2019 KAIST 로봇공학(박사)
2019~2020 한국전자통신연구원(ETRI) 연구원
2020~현재 계명대학교 로봇시스템공학과 조교수
관심분야: 이동로봇공학, SLAM, 컴퓨터비전