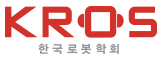
물체 변형 성능을 향상하기 위한 U-net 및 Residual 기반의 Cycle-GAN
© Korea Robotics Society. All rights reserved.
Abstract
The image-to-image translation is one of the deep learning applications using image data. In this paper, we aim at improving the performance of object transfiguration which transforms a specific object in an image into another specific object. For object transfiguration, it is required to transform only the target object and maintain background images. In the existing results, however, it is observed that other parts in the image are also transformed. In this paper, we have focused on the structure of artificial neural networks that are frequently used in the existing methods and have improved the performance by adding constraints to the exiting structure. We also propose the advanced structure that combines the existing structures to maintain their advantages and complement their drawbacks. The effectiveness of the proposed methods are shown in experimental results.
Keywords:
Deep learning, AI, Image-to-image translation, CNN, GAN1. 서 론
이미지-이미지 번역(Image-to-Image Translation)[1]은 이미 지를 활용하는 딥러닝의 응용 분야 중 하나로서, 인공신경망 의 입력과 출력 데이터가 모두 이미지 형태이다. 입력 이미지 의 도메인을 원하는 다른 도메인으로 변환하는 일을 수행하 며, 도메인을 어떻게 설정하느냐에 따라 다양한 응용이 가능 하다. 흑백 사진을 컬러 사진으로[2], 지도를 위성사진으로[1], 선화를 채색된 이미지로 변환[3]하는 등의 예시가 있다. 다루는 데이터의 형태가 이미지이기 때문에 합성곱 인공신경망 (Convolutional Neural Networks)을 기반으로 하여, 최근에는 딥러닝 분야에서 가장 활발하게 연구되고 있는 생성모델 중 하나인 GAN (Generative Adversarial Networks)[4]을 많이 사용 하고 있다. GAN은 판별(Discriminator)과 생성(Generator)을 담당하는 두 개의 인공신경망으로 구성되며, 학습 과정에서 두 개의 인공신경망이 서로 경쟁을 한다. 본 논문에서는 이미 지-이미지 번역의 일종인 물체 변형(Object Transfiguration)의 성능을 개선하고자 하였다. 물체 변형에서는 이미지 상의 특 정 물체 자체를 도메인으로 보고 다른 물체로 변형하는 일을 수행한다. 말을 얼룩말로 변형하거나 사과를 오렌지로 변형하 는 등의 예시를 들 수 있다. 본 논문에서는 이를 위해 GAN에 Cycle-Consistency 개념을 추가한 Cycle-GAN[5]의 형태를 사 용하였다. 일반적으로 특정 물체가 포함된 이미지에 대해 해 당 물체만 다른 물체로 변경된 이미지를 구하여 학습 데이터 로 사용하기는 어려운데, Cycle-GAN은 쌍이 맞지 않는 데이 터로도 학습이 가능하다. 즉, 변형할 두 도메인만 결정되면 각 데이터에 대응하는 상대 도메인의 데이터가 없어도 되기 때문 에 물체 변형에 적합한 형태이다. 이 때, 이미지 상에서 목표가 되는 물체만 정확하게 인식하고 해당 부분만 적절하게 변형하 는 것이 필요한데, 기존 결과에서는 목표가 되는 물체 이외의 부분에서도 변형이 생기는 현상을 관찰할 수 있다. 본 논문에 서는 GAN을 구성하는 인공신경망들 중 직접적으로 도메인 간의 변환을 학습하는 생성망의 구조에 초점을 맞추어 기존 방법의 한계점을 개선하고자 하였다. 즉, 기존 방법과 같이 생 성망이 상대 도메인의 전체 이미지를 생성하는 것이 아니라 특정 영역에 대한 마스크를 생성하여 물체 변형을 수행하도록 제약을 주었으며, 이를 통해 목표가 되는 물체에 집중하는 효 과를 얻을 수 있었다. 또한 기존에 사용된 생성망 구조와 이미 지-이미지 번역에서 자주 사용되는 구조를 분석하고 결합하 여 각각의 장점은 살리고 단점은 보완하는 새로운 형태의 구 조를 제안하였다. 실험에는 기존 Cycle-GAN에서 사용된 horse2zebra 데이터[6]를 사용하였으며, 본 논문의 목적에 따른 지표를 제시하여 정량적으로 성능을 평가하고자 하였다.
2. 관련 연구
2.1 GAN & Cycle-GAN
GAN (Generative Adversarial Networks)은 현재 딥러닝 분 야에서 가장 활발하게 연구되고 있는 생성모델 중 하나이며, 판별(Discriminator)과 생성(Generator)을 담당하는 두 개의 인 공신경망으로 구성되어 있다. 판별망은 입력된 데이터가 실제 데이터인지 생성망이 생성한 가짜 데이터인지를 판별하도록 학습하고, 생성망은 판별망을 속일 수 있는 데이터를 생성하 도록 학습한다. 이러한 경쟁 과정을 통해 이미지 생성을 학습 할 수 있으며, 이미지-이미지 번역에서도 좋은 성과를 보여주 고 있다. GAN은 수많은 변형 및 응용들이 존재하는데, 그 중 Cycle-GAN은 Cycle-Consistency의 개념이 추가된 모델이다. 데이터를 다른 도메인으로 변형하였다가 다시 본래의 도메인 으로 변형하였을 때 원본 데이터와 동일하게 유지되어야 한다 는 개념으로, 이 덕분에 쌍이 맞지 않는 데이터로도 학습이 가 능하다. 동일한 개념을 사용하는 연구로 Disco-GAN[7], Dual-GAN[8] 이 있다. 본 논문에서는 Cycle-GAN을 물체 변형 을 위한 큰 틀로 사용하고 내부에서 사용되는 인공신경망의 세부적인 구조에 초점을 맞추어, 구조적인 제약을 추가하고 기존 이미지-이미지 번역에서 빈번하게 사용되는 구조들을 결합함으로써 성능 향상을 얻고자 하였다.
2.2 Adversarial Loss
Cycle-GAN은 [Fig. 1]과 같이 두 쌍의 GAN으로 구성된다. 변환을 수행할 두 도메인이 결정되면, 각각의 GAN을 통해 두 도메인 간의 양방향 변환이 동시에 학습된다. 판별망과 생성 망의 적대적인 구도를 위한 기본적인 손실함수(Loss)는 식 (1) 과 같고, 서로의 입장에서 이를 최적화하기 위해 경쟁한다.
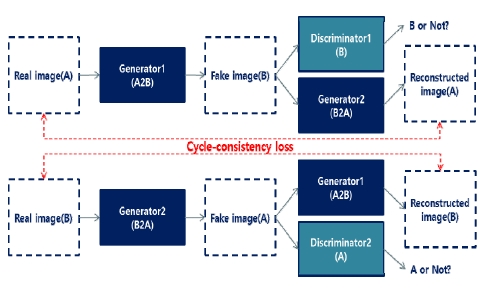
Cycle-GAN: There are two domains (A, B) to perform object transfiguration, and a pair of generator and discriminator for learning transfiguration from each domain to a relative domain. Cycle-consistency loss is caused by the difference between the real image and the reconstructed image transformed back into original domain
(1) |
여기서 판별망 Dy는 y도메인의 실제 이미지 또는 가짜 이 미지 를 입력받아 y도메인의 실제 데이터가 맞는지 를 판별하고, 생성망 는 x도메인의 이미지를 입력으로 받 아서 판별망 Dy를 속일 수 있는 y도메인의 이미지를 생성한 다. 두 도메인 사이에서 이러한 매핑을 학습하므로 각각 와 가 존재한다.
2.3 Cycle-consistency Loss
Cycle-consistency Loss는 상대 도메인으로 변형한 이미지 를 다시 원래의 도메인으로 변형한 이미지와 원본 이미지 간 의 차이에서 발생하며, 식 (2)와 같이 계산된다.
(2) |
여기서 A, B는 각 도메인에서의 실제 이미지 데이터이다. 손실함수에 이를 추가함으로써 Adversarial loss만 사용했을 때보다 입력과 출력 간의 매핑에 제약이 생긴다.
2.4 Full Objective
최종적으로 Cycle-GAN을 통해 최적화할 목적함수는 식 (3) 과 같다.
(3) |
여기서 λ는 각 loss들 간의 중요도를 조절하기 위한 가중치 이다. 이 목적함수를 판별망들은 최대화, 생성망들은 최소화 하기 위해 서로 경쟁하며 학습하고, 이 과정을 통해 두 도메인 간의 변환을 생성망에 학습시킬 수 있다.
3. 제안하는 방법
본 논문에서는 물체 변형 성능을 향상시키기 위해 생성망 의 세부 구조에 초점을 맞추었다. 첫 번째로, 간단한 구조적 제 약(Input-residual)을 추가하고, 두 번째로는 기존에 사용되던 구조들의 특성을 분석하고 이들을 결합하여 장점은 유지하고 단점은 보완할 수 있는 구조(ω-net)를 제안한다. 최종적으로는 이 두 가지를 조합하여(ω-net과 Input-residual의 조합) 본 논문 의 목표를 달성하고자 한다.
3.1 구조적 제약을 추가한 Input-residual
이미지 상의 물체가 변형되기 위해서는 목표가 되는 물체 에 해당하는 특정 영역의 픽셀 값들이 조정되어야 한다. 본 논 문에서는 이러한 아이디어를 생성망에 제약으로 주고자 하였 다. [Fig. 2]와 같이 생성망의 입력과 출력을 더하여 결과를 내 도록 덧셈 연결을 추가함으로써 이러한 제약을 주었다.
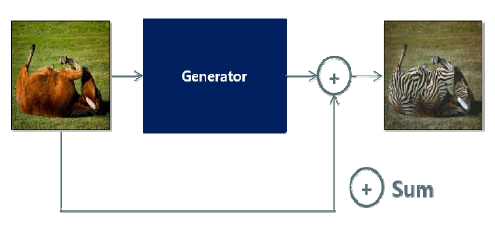
Input-residual: We added the connection to make a result by adding the input and output of the generator. This constrains the object transfiguration to be performed through mask generation
이 연결을 통해 생성망은 변형된 전체 이미지를 생성하는 것이 아니라 입력 이미지에 더해질 마스크를 생성하여 물체 변형을 수행하도록 제약된다. 이미지의 생성 과정을 식 (4)와 같이 변경한 것으로 볼 수 있다.
(4) |
여기서 a는 생성망으로 들어가는 입력 이미지, b는 생성된 이미지이다. 이 때, G(a)를 입력에 더할 마스크로 볼 수 있다. 인공신경망의 전체 입력과 출력에 대한 덧셈연산을 추가했을 뿐이며, 형태는 Residual Block과 동일하다. 이를 통해 물체 변 형을 수행할 때 목표가 되는 물체에 집중하는 효과를 얻을 수 있었다.
3.2 기존의 Residual-net과 U-net 분석
딥러닝에서 Residual[9,10]은 인공신경망이 깊어질수록 학습 이 잘 되지 않는 점을 개선하기 위한 방법으로 연구되었다. [Fig. 3]에서처럼 Residual Block은 층을 건너뛰는 덧셈 연결을 통해 입력과 출력의 차이를 학습하게 되며, 기울기의 흐름이 원활해져 깊은 인공신경망도 학습이 가능해진다. 이러한 구조 는 입력에 어떤 작은 변화를 생성하여 더하는 형태로도 볼 수 있는데, 이러한 특성으로 인해 이미지-이미지 번역에서는 변 환기(Transformer)의 역할로 자주 사용되고 있다. Cycle-GAN 의 생성망 역시 Residual Block을 기반으로 구성되어 있다[11].
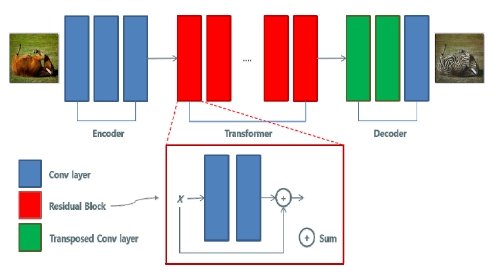
Residual-net: The composition of the residual-net can be roughly divided into an encoder, a decoder, and a transformer. Among them, the residual block is used as a transformer
[Fig. 3]의 구조는 크게 인코더(Encoder)와 변환기(Transformer), 디코더(Decoder) 부분으로 나눌 수 있다. Residual Block이 담당하고 있는 변환기 부분을 제외하면 인코더와 디 코더는 비교적 얕은 층으로 구성되어 있다. 합성곱 인공신경 망은 층이 깊어질수록 좀 더 상위 레벨의 추상화된 특징을 추 출할 수 있으며, 이러한 과정에서 동반되는 다운샘플링을 통 해 크기, 위치 등에 대해 어느 정도 불변의 특성을 가지게 된다. 하지만 인코더-디코더 단이 얕다는 것은 물체를 정확하게 인 식해야 하는 경우에는 단점이 될 수 있다. 이에 따라 실제 변형 이 잘 된 예시들에서도 물체 이외의 부분 또한 변형이 발생하 는 현상을 관찰할 수 있다.
U-net[12]도 이미지-이미지 번역에서 자주 사용되는 구조 중 하나이며 의료영상 분할을 위해 제안된 구조이다. 앞서 기술 한 것처럼 다운샘플링은 합성곱 인공신경망이 어느 정도 불변 한 특성을 가지는데 기여하는 반면, 정확한 공간적인 정보의 손실을 야기하기도 한다. 이미지 분할(Image Segmentation)에 서는 정확한 공간적인 정보가 필요하기 때문에 이를 보완하고 자 인코더와 디코더 사이에 연결을 추가한 것이 U-net의 핵심 이다. [Fig. 4]에서와 같이 디코딩 때 인코더 단에서 나온 특징 정보도 같이 활용할 수 있도록 연결된다. 즉, 공간적인 정보를 유지하면서도 층을 더 깊게 쌓을 수 가 있고, 이러한 구조가 인 코딩-디코딩 측면에서는 Residual-net보다 유리하다고 볼 수 있다. 하지만 반대로 Residual Block을 사용하지 않기 때문에 변환의 개념이 빈약하다.
3.3 Residual Block을 추가한 ω-net
본 논문에서는 [Fig. 5]와 같이 Residual-net과 U-net을 결합 하여 서로의 장점은 살리고 단점은 보완할 수 있는 형태의 구 조를 제안한다. 인코딩-디코딩 측면에서 좀 더 유리한 U-net의 구조를 기본 골격으로 하였으며, 인코더와 디코더 사이에 Residual Block을 거치도록 추가하여 변환의 개념을 보완하였 다. Residual-net에서처럼 특정 해상도에서만 Residual Block 을 통한 변환을 거치는 것이 아니라 여러 해상도에서 이러한 과정을 거치게 되는데, 이는 컴퓨터 비전 분야에서 자주 쓰이 는 기법과도 유사하다. 또한 같은 크기의 Residual Block이라 하더라도 더 낮은 해상도에서 연산을 수행하기 때문에 구성에 따라 연산량 절감 효과도 얻을 수 있다. Residual-net에 비해 더 큰 해상도에서의 연산도 추가되지만 큰 해상도에서는 비교적 적은 채널 수를 사용하기 때문에 연산량을 줄일 수 있다.
4. 실 험
4.1 실험 방법
실제 구현에서는 좀 더 안정적인 것으로 알려져 있는 LSGAN[13]의 Adversarial Loss를 사용하였으며, Cycle-GAN의 최적화를 위해 Adam을 사용하였다. 이 때, 학습률은 0.0002로, beta는 각각 0.5와 0.999로 하였으며, 배치 크기는 4로 하였다. 총 150 세대(epoch) 동안 판별망과 생성망을 각각 한 번씩 갱 신하였고, 100세대 이후부터는 0까지 선형적으로 학습률을 감쇄시켰다. 이미지의 크기는 256×256으로 고정하였고, 30×30 크기의 패치 형태 판별망(Patch Discriminator)을 사용 하였다. 이때, 50장 크기의 이미지 풀[14]을 두었다. 위와 같은 요소들은 모두 동일하게 적용하고 생성망의 구조를 변경해가 며 실험을 진행하였다.
4.2 데이터 세트
실험에는 기존 Cycle-GAN에서 사용되었던 horse2zebra 데 이터[6]를 사용하였다. 본 논문의 실험과 관련성이 낮은 데이터 들은 일부 삭제하여 총 말 816장, 얼룩말 948장으로 학습 데이 터를 구성하였다. 테스트 데이터 세트로는 Weizmann Horses 데이터 세트[15]의 말 이미지 328장을 사용하였다. 이는 정략적 인 지표를 측정하기 위한 것으로서 다음 절에서 설명한다.
4.3 배경 유지 성능을 평가하기 위한 정량적 지표
생성모델이 생성한 데이터를 정량적으로 평가하는 것은 어 려운 일이다. 예를 들어 말이 얼룩말로 얼마나 잘 변형되었는 지는 주관적인 판단으로 평가되기 때문이다. 따라서 이미지- 이미지 번역에서는 주로 Amazon Mechanical Turk (AMT)를 이용한 정성적인 설문조사가 사용된다. 하지만 배경 유지 성 능은 색차 계산을 통해 정량적인 평가가 가능하다. Weizmann Horses 데이터 세트에는 328장의 말 이미지와 함께 말 부분만 분할되어있는 Segmentation map이 존재한다. 이를 통해 배경 부분만 분할할 수 있으며 분할된 배경 부분에 대해 변형 전 후 의 색차를 계산함으로써 정량적인 평가를 할 수 있다. 색차 계 산에는 인간의 눈에 의한 색차 인식을 가능한 가깝게 수학적 으로 재현하기 위해 만들어진 Lab 색 공간을 사용하였다. 이 때, 색차는 식 (5)와 같이 계산된다. (CIELAB Delta E* - CIE76)
(5) |
L, a, b는 Lab 색공간의 각 채널로서, L은 밝기를, a는 빨간 색과 초록색 중 어느 쪽으로 더 치우쳐져 있는지를, b는 노란 색과 파란색 중 어느 쪽으로 더 치우쳐져 있는지를 나타낸다. 각 픽셀에 대해 색차를 계산한 후, 식 (6)과 같이 전체 이미지 중 색차가 일정한 문턱 값 이하로 유지되는 픽셀의 비율을 지 표로 사용하였다.
(6) |
여기서 h, w는 각각 이미지의 높이와 너비를 나타낸다. 전 체 테스트 데이터들에 대해 식 (6)을 적용하고, 평균값을 구하 여 정량적인 평가를 하였다. 문턱 값은 CIE76 색차 값에 따른 사람의 인지에 대한 조사를 참고하여 0~10으로 변경해가며 평가를 진행하였다. [Table 1]은 이러한 조사의 예시로서, 조사 및 규격에 따라 조금씩 차이가 있다.
4.4 정성적 평가
Input-residual을 추가한 후 생성망의 동작이 마스크를 생성 하도록 변경되는 것을 [Fig. 6]을 통해 확인할 수 있다. 여기서 가장 왼쪽의 이미지는 입력 이미지이고, Residual-net을 통해 물체 변형한 결과가 두 번째 이미지이다. 세 번째 이미지는 Residual-net에 Input-residual 연결을 추가한 후 생성망이 생성 한 마스크를 나타내며, 입력 이미지와 더해져 네 번째의 최종 결과를 만들어 낸다. 네 번째 이미지가 두 번째 이미지에 비해 배경이 더 잘 유지되는 것을 확인할 수 있다.
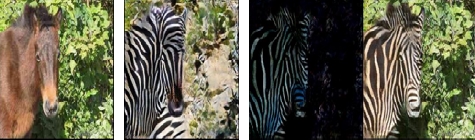
Input-residual: The leftmost image[6] is the input image, and the second image is the result of object transfiguration through residual-net. The third image shows the mask generated by the generator after connecting input-residual to residual-net. This mask is added to the input image and the fourth image is the final result. We can see the background is better maintained with input-residual
[Fig. 7]은 Residual-net과 ω-net에 Input-residual을 적용하고 결과를 비교한 예시이다. 두 번째 열의 결과들과 같이 Residual-net에 Input-residual을 추가하였음에도 여전히 배경 에 노이즈가 생기는 것을 살펴볼 수 있다. 세 번째 열의 결과들 은 이러한 현상이 ω-net을 통해 개선됨을 보여준다.
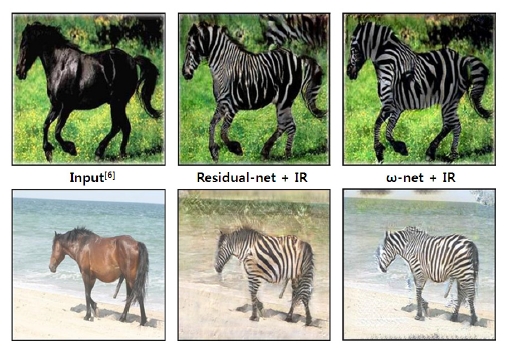
Residual-net vs. ω-net: These are some examples of comparing the results of residual-net and ω-net. Input-residual is applied to both neural networks. In residual-net, we can see through the second column images that background noise is still generated even though input-residual is applied. In third column images, we can see this tendency is relatively improved by ω-net
[Fig. 8]은 U-net과 ω-net에 Input-residual을 적용하고 결과 를 비교한 예시이다. 두 번째 열의 결과들과 같이 U-net에서는 대상 물체가 잘 변형되지 않지만 세 번째 열의 결과들과 같이 ω-net에서는 잘 변형됨을 확인할 수 있다.
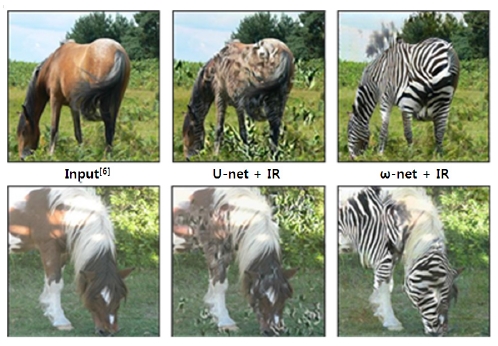
U-net vs. ω-net: These are some examples of comparing the results of u-net and ω-net. Input-residual is applied to both neural networks. In u-net, there are some examples that do not transfigurate well like the second column images, while we can see these examples can be transfigurated well by ω-net (third column images)
4.5 배경 유지에 대한 정량적 평가
[Fig. 9]는 328장의 테스트 데이터 세트에 대하여 4.3절에서 제시한 지표를 통해 각 구조들을 배경 유지 관점에서 평가한 결과를 나타낸다. 즉, 목표가 되는 물체가 얼마나 잘 변형되었 는지를 평가하는 것이 아니라 배경이 얼마나 잘 유지되는지만 평가한다. Input-residual을 추가하기 전의 각 구조들은 배경 유 지의 측면에서 모두 낮은 성능의 결과를 보이지만, Inputresidual을 추가한 후에는 각 구조에서 모두 배경 유지 성능이 개선됨을 볼 수 있다. 이때, U-net과 Input-residual의 조합이 배 경 유지 측면에서 수치적으로 가장 높은 성능을 보이고 있지 만 [Fig. 10]과 같이 변형 자체가 상대적으로 크게 미흡하다는 문제점을 확인할 수 있다. 즉, U-net과 Input-residual의 조합이 배경을 잘 유지하는 것처럼 보이지만 이는 이미지 변형 자체 가 잘 수행되지 않기 때문에 배경 또한 변형이 거의 발생하지 않은 것이다. 따라서 본 논문의 목표에 부합한다고 할 수 없다. 이에 반해 제안하는 방법인 ω-net과 Input-residual의 조합은 물체를 잘 변형하면서도 Residual-net과 Input-residual의 조합 에 비해 배경이 더 잘 유지된다는 것을 확인할 수 있다.
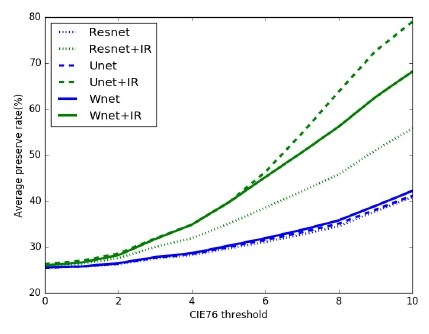
This graph shows the result of evaluating background maintenance for each structure through the metric presented in this paper. In all three structures, we can see that the background maintenance are improved through input-residual. Here, the combination of u-net and input-residual shows the highest performance, but there is a problem that the transfiguration is relatively insufficient ([Fig. 10])
5. 결 론
본 논문에서는 물체 변형을 수행할 때 물체 이외의 부분도 같이 변형되는 기존 방법의 문제를 개선한 새로운 생성망 구 조를 제안하였다. 직접적으로 변형을 담당하는 인공신경망에 제약을 추가하고, 기존 구조들을 결합하여 세부적인 구조를 변경함으로써 배경을 유지하면서 물체만 변형될 수 있도록 하 였다. 또한 정량적인 평가를 위한 지표를 제시하여 기존 방법 과 비교하고 성능을 검증하였다. 하지만 본 논문에서 제시한 지표는 배경 유지라는 목표를 평가하기 위한 것으로서 물체의 변형 자체가 잘 되었는지를 판단하기 위한 것은 아니다. 향후 이를 평가하고 검증할 수 있는 방법에 대한 연구가 필요하며, 지속적인 실험을 통해 더욱 최적화하는 것이 필요하다.
Acknowledgments
This work was supported by the Convergence Technology Development Program for Bionic Arm through the National Research Foundation of Korea funded by the Ministry of Science, ICT and Future Planning, South Korea, under NRF-2015M3C1B2052811.
References
-
P. Isola, J-Y. Zhu, T. Zhou, A.A. Efros, Image-to-image translation with conditional adversarial networks, 2017 IEEE Conference on Computer Vision and Pattern Recognition, Honolulu, USA, p5967-5976, (2017).
[https://doi.org/10.1109/cvpr.2017.632]
- R. Zhang, P. Isola, A.A. Efros, Colorful image colorization, European Conference on Computer Vision, Amsterdam, Netherlands, p649-666, (2016).
- Preferred Networks, PaintsChainer, (2018).
- I. Goodfellow, J. Pouget-Abadie, M. Mirza, B. Xu, , Generative adversarial nets, 28th Annual Conference on Neural Information Processing Systems, Montreal, Canada, p2672-2680, (2014).
-
J-Y. Zhu, T. Park, P. Isola, A.A. Efros, Unpairedimage-to-image translation using cycle-consistent adversarial networks, 2017 IEEE International Conference on Computer Vision, Venice, Italy, p2242-2251, (2017).
[https://doi.org/10.1109/iccv.2017.244]
-
O. Russakovsky, J. Deng, H. Su, J. Krause, S. Satheesh, S. Ma, Z. Huang, A. Karpathy, A. Khosla, M. Bernstein, A.C. Berg, L. Fei-Fei, ImageNet Large scale visual recognition challenge., Int. J. Comput. Vis., (2015), 115(3), p211-252.
[https://doi.org/10.1007/s11263-015-0816-y]
- T. Kim, M. Cha, H. Kim, J.K. Lee, J. Kim, Learning to discover cross-domain relations with generative adversarial networks, International Conference on Machine Learning, Sydney, Australia, p1857-1865, (2017).
-
Z. Yi, H. Zhang, P. Tan, M. Gong, Dualgan: Unsupervised dual learning for image-to-image translation, IEEE International Conference on Computer Vision, Venice, Italy, p2868-2876, (2017).
[https://doi.org/10.1109/iccv.2017.310]
-
K. He, X. Zhang, S. Ren, J. Sun, Deep residual learning for image recognition, 2017 IEEE International Conference on Computer Vision, Las Vegas, USA, p770-778, (2016).
[https://doi.org/10.1109/cvpr.2016.90]
-
K. He, X. Zhang, S. Ren, J. Sun, Identity mappings in deep residual networks, European Conference on Computer Vision, Amsterdam, Netherlands, p630-645, (2016).
[https://doi.org/10.1007/978-3-319-46493-0_38]
-
J. Johnson, A. Alahi, L. Fei-Fei, Perceptual losses for real-time style transfer and super-resolution, European Conference on Computer Vision, Amsterdam, Netherlands, p694-711, (2016).
[https://doi.org/10.1007/978-3-319-46475-6_43]
-
O. Ronneberger, P. Fischer, T. Brox, U-net: Convolutional networks for biomedical image segmentation, International Conference on Medical Image Computing and Computer Assisted Intervention, (2015), Munich, Germany, p234-241.
[https://doi.org/10.1007/978-3-319-24574-4_28]
-
X. Mao, Q. Li, H. Xie, R.Y.K. Lau, Z. Wang, S.P. Smolley, Least squares generative adversarial networks, 2017 IEEE International Conference on Computer Vision, Venice, Italy, p2813-2821, (2017).
[https://doi.org/10.1109/iccv.2017.304]
-
A. Shrivastava, T. Pfister, O. Tuzel, J. Susskind, W. Wang, R. Webb, Learning from simulated and unsupervised images through adversarial training, 2017 IEEE Conference on Computer Vision and Pattern Recognition, Honolulu, USA, p2242-2251, (2017).
[https://doi.org/10.1109/cvpr.2017.241]
-
E. Borenstein, S. Ullman, Combined top-down/bottom-up segmentation., IEEE Trans. Pattern Anal. Mach. Intell., (2008), 30(12), p2109-2125.
[https://doi.org/10.1109/tpami.2007.70840]
- W. Mokrzycki, M. Tatol, Color difference ΔE - A survey., Machine Graphic and Vision, (2011), 20(4), p383-411.